The past year brought C2SMART more growth, progress, and partnership-building...
Read MoreHow do we know whether a section of the roadway is at high safety risk?
Historically, the answer has been relatively straightforward: we count the number of crashes at that location over a period of time and compare it to other roadway sections. If the roadway section of our interest contains a high number of crashes, we then decide what can be done, if anything, to lower the number of crashes.
This method has been the go-to method for safety evaluation for decades, and with good reason. The number of crashes (or other crash-based safety measures) at a roadway section is a fairly straightforward piece of evidence regarding its relative safety risk level. There’s only one problem with it, which is that in order to obtain this data, we have to wait for crashes to happen.
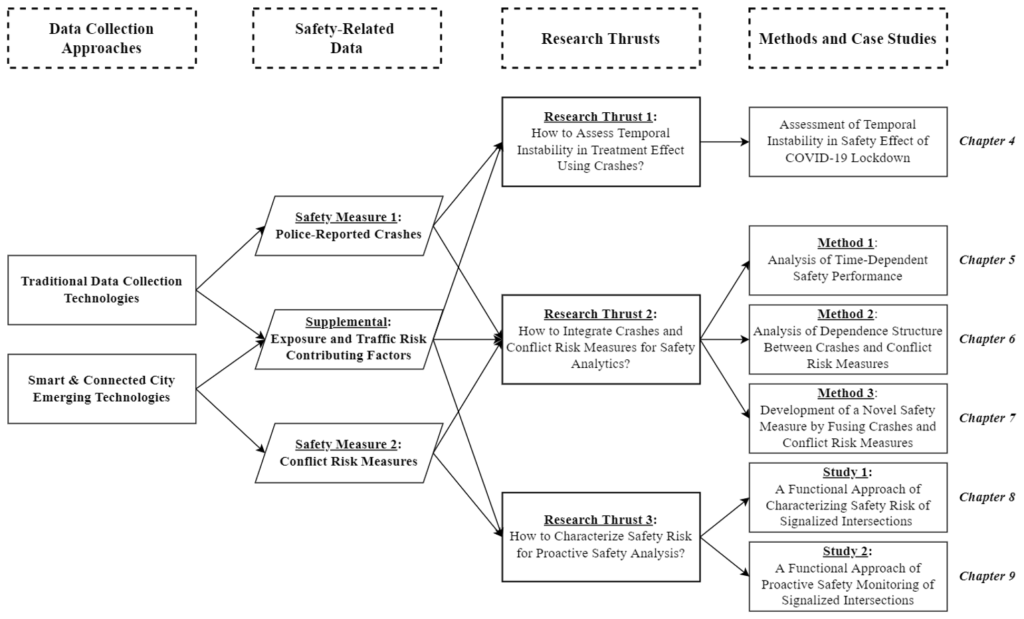
Researchers at New York University’s Urban Mobility and Intelligent Transportation Systems Laboratory (UrbanMITS) led by Professor Kaan Ozbay, part of the C2SMART University Transportation Center, have been studying solutions to this problem using innovative techniques made possible by the recent explosion in big data in transportation systems. The lab, a multi-modal transportation infrastructure research and education facility, uses emerging technology to improve transportation infrastructure for increased mobility, safety and comfort.
Di Yang, an UrbanMITS @ C2SMART researcher in this area, recently received his PhD from NYU’s Tandon School of Engineering.
“There is a good reason why traffic crashes have been used so widely in safety evaluation for so many years,” says Dr. Yang. “It was probably the only key safety measure that was feasible to collect on a large scale using traditional data collection techniques. But that’s changing now. With all of the emerging Intelligent Transportation Systems technologies – Connected and Autonomous Vehicles, smartphones, instrumented probe vehicles, traffic surveillance cameras, etc. – we are presented with incredible opportunities to use big data collected from these emerging technologies to evaluate safety risk before crashes occur.”
Part of UrbanMITS @ C2SMART’s work builds on these efforts by focusing on how to evaluate safety risk through the use of the so-called “traffic conflict data.” The term “traffic conflict” is often used to describe any scenario where road users need to take evasive actions to avoid a potential collision; for example, a driver swerving abruptly or slamming on the breaks. Traditionally, traffic conflict data would have been, if not impossible, extremely time-consuming and expensive to collect. New, emerging technologies, however, make the collection and extraction of traffic conflict data much easier.
“When we are using crash data, we have to wait for crashes to happen – essentially, we are waiting for people to get injured or killed before safety risk can be evaluated. So from this perspective, the use of crash data is often regarded as a reactive approach,” Yang explains. “But the use of traffic conflict data is a proactive approach, because no one has to get injured or killed in order to evaluate safety.”
So, the key question lies in how to properly use traffic conflict data to facilitate proactive safety evaluation and management. As part of his PhD dissertation, he proposed a novel statistical and data-driven approach that can properly characterize the safety risk embedded in traffic conflict data.
Common practices in traffic conflict data analysis often aggregate traffic conflict events at certain temporal and spatial levels, such as every several minutes or for each intersection approach. However, since traffic conflict events are extracted from advanced emerging technologies, traffic conflict events possess very detailed spatio-temporal information. As a result, aggregating traffic conflict events at certain data aggregation levels may not be ideal to fully reveal the safety risk patterns embedded in traffic conflict data and may result in the loss of valuable information.
In other words, the information is there; we just need to learn how to properly capture it. By proposing to model safety risk embedded in traffic conflicts as functions with respect to time, this novel approach reveals more detailed and interesting safety risk patterns that were rarely discovered before. The proposed functional approach also produces promising results in terms of detecting safety-related anomalies which provide agencies and engineering companies with the opportunity to improve safety operations on multiple levels.
Yang believes this novel functional approach is just the starting point, and has the potential to provide three particularly salient points of departure for future proactive safety evaluation and management:
- Designing more targeted and real-time safety alerts to drivers;
- Calibration of micro-simulation models for safety evaluation; and
- Optimization of signal timing accounting for safety.
Transportation technology is evolving rapidly, and shows no sign of slowing down. It has helped produce incredible advancements in how we travel; but equivalent steps forward in safety evaluation still requires more attention.
“There is no longer any reason to wait for fatalities or injuries to happen,,” says Yang. “We can learn just as much, or even more, from when collisions don’t happen–and use that knowledge to ensure they never do.”
Di Yang received his PhD from the Department of Civil and Urban Engineering at New York University in 2022 is an incoming tenure track Assistant Professor in the Department of Transportation & Urban Infrastructure Studies at Morgan State University. His research focuses on developing novel statistical and data-driven methods at the intersection of transportation safety, connected and autonomous vehicles, human factors, and shared mobility. His research contributions have led to 26 publications in premium journals and conferences, featuring 11 first-author papers, and recognized by the prestigious Institute of Transportation Engineers (ITE) Northeastern District Daniel B. Fambro Student Paper Award in 2021.
Before joining NYU, he obtained his Bachelor’s degree at Beijing Jiaotong University. His research interests include traffic safety, connected and autonomous vehicles, and surrogate safety measures.
Related Posts
C2SMARTER FDNY Collaboration Included in Popular Science’s 50 Greatest Innovations of 2024
December 9, 2024 The AI-reinforced Digital Twin focused C2SMARTER project,...
Read MoreC2SMARTER at TRB 2025
The Transportation Research Board (TRB) 102nd Annual Meeting was held...
Read MoreC2SMARTER Announces 2024-2025 Research Agenda
In 2025, C2SMARTER is focused on reducing congestion and ensuring...
Read More