Overview
Challenges in Vehicle Rebalancing for Car Sharing Services
Car sharing is a smart mobility paradigm where a population of users share usage of the vehicles. This can be done through booking vehicles for specific time slots from specific locations that are returned to the same locations (two-way car sharing), such as Zipcar, or through the one-way car sharing business model, which allows users to reserve a vehicle and drop it off at any other station (station-based) or location (free floating) within the system. Examples include Car2Go. Car sharing has been shown to be a very sustainable operating model for cities; Martin and Shaheen (2016) showed that a single Car2Go vehicle can effectively remove 7 to 11 privately owned passenger cars from the road.
In New York, the largest megacity in the U.S., the competition for car sharing services is on the rise. Car2Go has a fleet of 550 vehicles, while BMW recently launched a 250-vehicle fleet via its ReachNow division in the same Brooklyn region (Flamm, 2016). The figure below shows the coverage areas of three car sharing services in New York City (NYC): Car2Go, Zipcar, and ReachNow. Both Car2Go and ReachNow are free floating, one-way car sharing systems. Other carsharing services have been developed in New York State as well, such as Ithaca Carshare (Ithaca Carshare, 2011) and an EV car share pilot in Buffalo funded by NSERDA.
Vehicle rebalancing is one of the primary challenges of operating a car sharing service. Potential customers may balk from using the service if there is no available vehicle nearby, or no parking location available near the destination. Staff (or incentivizing schemes for travelers) is needed to relocate idle vehicles to make sure there is sufficient demand throughout the system. Recent findings from simulation tests suggest dynamic rebalancing based on queueing networks has promise in helping solve this problem.
Rebalancing in Electric Vehicle Car Sharing Systems
Rebalancing is further hampered in an electric vehicle (EV) environment. Limited EV infrastructure and long charging times make balancing EV availability by service providers or users much costlier to operate. For example, Car2Go had to switch their EVs in San Diego to gasoline technology because of lack of supporting infrastructure, and eventually shut down service there. None of the shared services in NYC are EV-based, as the shared services all operate outside Manhattan for the cheaper parking options and demand, but there is limited charging infrastructure in the other boroughs (see Fig. 2). Car sharing operations face a drop in passenger demand due to the inconvenience of charging if they switch to EV operations.
The problem is that shared EV operators have TWO rebalancing problems: one to meet customer demand and one to ensure charged vehicles are removed from charging stations while low-charge vehicles are swapped in. Free floating systems reduce the need for rebalancing, but a free-floating system operating with EVs introduces a whole new set of balancing requirements that have not been well studied. Very few systems have been developed to consider both electric vehicle charging and the need to balance vehicles, and all of those have only considered station-based operations. Station-based operations lead to lower demand for the service (due to increased detours), and hasvea high cost since every vehicle would need a parking and charging location. In NYC, this can be especially challenging, as noted by Car2Go, which collects thousands of parking tickets a year. Developing an effective design solution will make it feasible for smart mobility companies like ReachNow to plan how to expand their current free-floating systems and consider implementing EV fleets with evaluation of different charging location plans.
Related Media
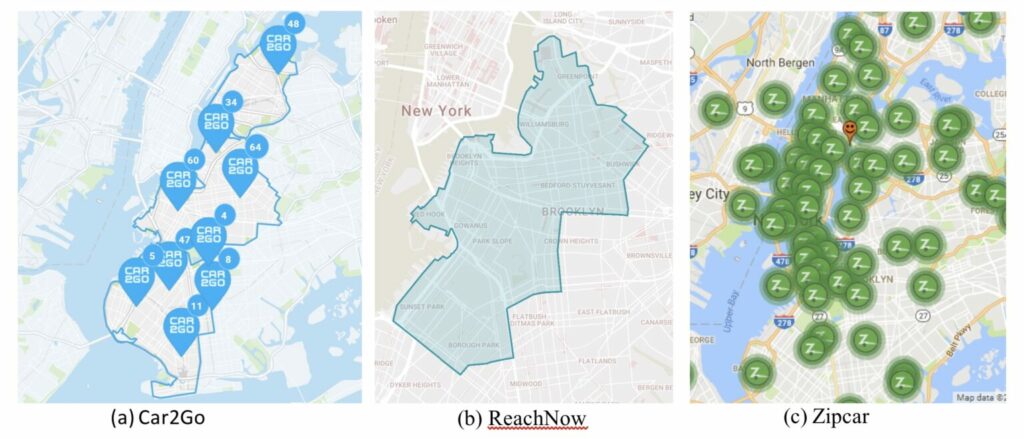
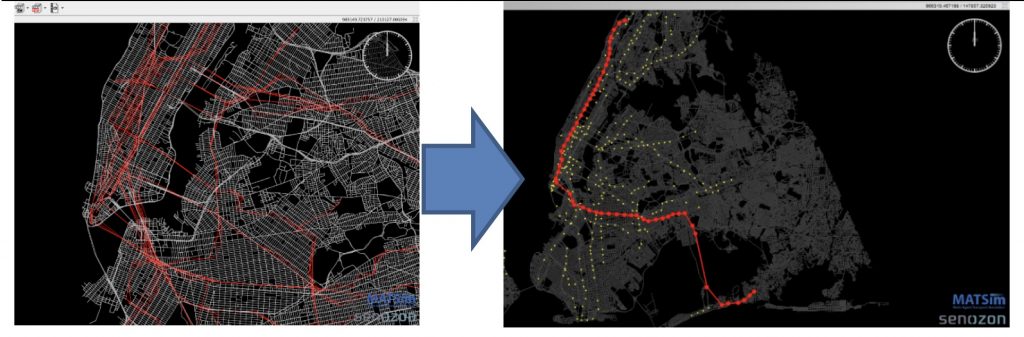
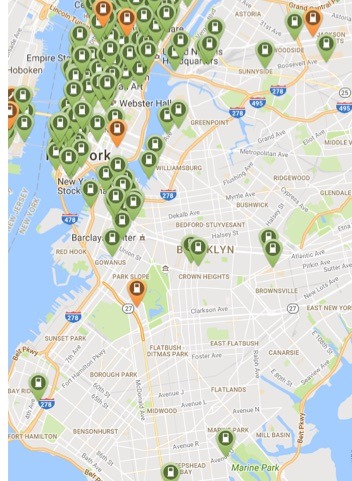
Research Objectives
The research team aims to test a new queueing network-based dynamic rebalancing strategy in test cases provided by ReachNow in Brooklyn, NY. The strategy can operate in a non-EV as well as an EV setting, so computational experiments for both will be conducted.
In addition, the researchers will develop a MATSim agent model of the study area in NYC, calibrate it based on household travel survey data from NYMTC, Openstreetmap, traffic data from NYCDOT, and transit schedules from GTFS. Example screenshots of the uncalibrated base model are shown in Fig. 3 above.
The model will be calibrated with the non-EV carsharing fleet data from ReachNow. The simulation tool will be modified to embed the new rebalancing algorithm that the researchers are developing. Based on the data, the research team can then evaluate non-EV operations with the proposed rebalancing strategy and compare social welfare measures. EV operating data will not be available at the time of this project to evaluate an EV fleet with MATSim.
The team plans to end the project with a workshop for invited guests held jointly with Don Mackenzie’s research group from University of Washington so the two teams can share findings from their car sharing projects.