Overview
Electric vehicles (EVs) generally lead to a reduction in greenhouse gas emissions and have the potential to reduce our dependency on fossil fuels and increase the penetration of renewable sources of energy. Further, new mobility services, like carsharing in general and free-float carsharing (FFCS) in particular, have the potential to reduce the need for car ownership and complement transit, ultimately reducing vehicle miles traveled. Electric free-float carsharing (eFFCS) is the amalgamation of the two concepts, which promotes emission-free mobility, while providing the flexibility of owning and operating the vehicle only between and during the points of travel. The pay-per-minute-use subscription model and features like park anywhere within service area make FFCS quite attractive for the environmental and economically conscious, ever-mobile, smartphone-savvy population of the 21st century.
Presently, due to slow recharging times of electric vehicles compared to gasoline-fueled vehicles, a charging event represents a constraint on trips and requires staff labor to relocate the free-floating vehicle to a charging station. This relocation leads to a high operational expenditure and unreliable downtimes. This study aimed to develop a demand model for an eFFCS service in the City of Seattle, which can increase the feasibility of eFFCS by reducing the cost of relocation by optimally locating the charging stations near the areas of heavy usage and real-time control to minimize manual relocation. The model consists of a destination model predicting end location given the start location, and a duration model predicting the dwell time after a trip at a particular location. The researchers uses trip data provided by ReachNow to develop the destination model and data from the car2go API for the duration model.
The long-term goal of this work is to integrate strategic and tactical management of the vehicle-infrastructure system to support electrified shared mobility using these models of trip requests and destinations. The researchers have developed stochastic models of shared car usage patterns, comprising traveler destination choices and vehicle dwell times conditional on time of day and day of the week, and have compared classical approaches (e.g. proportional hazards model, multinomial logit) with machine learning alternatives (e.g. neural networks, Markov chains).
Future research can build on this foundation by developing algorithms that integrate real-time information on state of charge, customer demand trends, and infrastructure availability to maximize shared electric mobility at minimal cost to the operator. This tactical control model can then be built into a strategic infrastructure network design model that determines the locations, charging rates, and number of plugs for each charging station.
This work is expected to support the design of cost-effective charging systems to support electrified shared mobility. This will allow shared mobility services to be electrified at a lower cost, increasing adoption of the technology, saving travelers and businesses money, and reducing noise and air pollution in cities.
Research Objectives
The primary deliverable for this project is a report and associated software documenting and implementing a system of models for simulating the stochastic movements of electric, shared vehicles around Seattle. The researchers created a trajectory synthesizer that combines the destination and duration models developed during this project, which is available online as an interactive web app. The tool allows users to select a number of trips and a block of time, then predicts the trajectory based on a clicked start position on the map.
Related Media
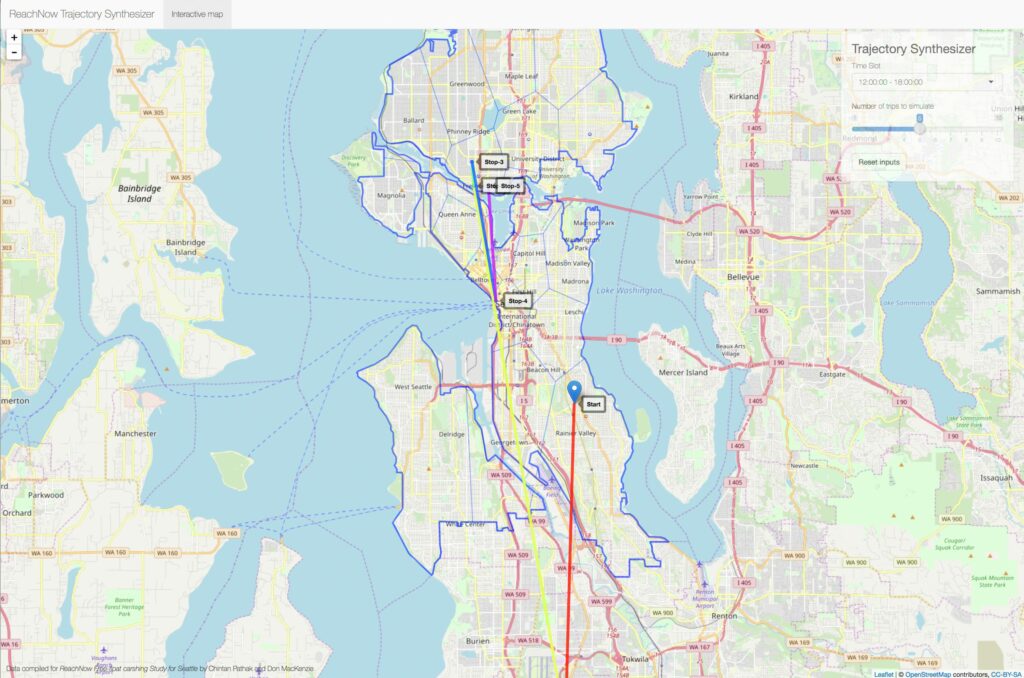
The research team developed a trajectory synthesizer that allows for selection of number of trips and time block during the day and then predicts the trajectory of a carshare vehicle based on a given start location.