C2SMARTER's innovative computer vision solutions leverage deep learning tailored to urban environments, enabling real-time object detection and tracking to improve mobility and safety for vehicles and vulnerable road users.
By building on existing resources and handling diverse video data—even with low-resolution and discontinuous videos—we provide new performance and risk indicators that are adaptable and scalable for large-scale implementation.
How Does it Work?
Our innovative approach to transportation monitoring, planning, and management uses the power of deep learning-based computer vision techniques using existing CCTV camera infrastructure, portable cameras, and in-vehicle cameras from connected and automated vehicles (CAVs). By detecting and tracking objects in real time, we aim to fill critical data gaps for vulnerable road users, vehicles, and infrastructure. What sets us apart is our data-centric localized training for each use case, tailored to meet the unique needs of agencies and transportation systems.
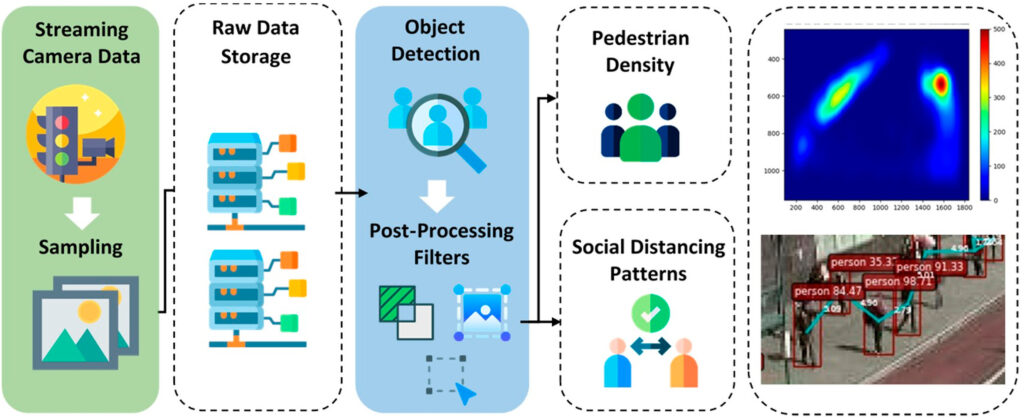
The following selection of articles provides more information on methodology.
Pedestrian detection: F. Zuo, J. Gao, A. Kurkcu, H. Yang, K. Ozbay, and Q. Ma. (2021). Reference-free video-to-real distance approximation-based urban social distancing analytics amid COVID-19 pandemic. Journal of Transport & Health, Vol 21.
Work zone detection: F. Zuo, J. Gao, K. Ozbay, Z. Bian, and D. Zhang. (2023). Urban Work Zone Detection and Sizing: A Data-Centric Training and Topology-Based Inference Approach. IEEE 26th International Conference on Intelligent Transportation Systems, Bilbao, Spain.
Parking: J. Gao, F. Zuo, K. Ozbay, O. Hammami, and M. Barlas. (2022). A new curb lane monitoring and illegal parking impact estimation approach based on queueing theory and computer vision for cameras with low resolution and low frame rate. Transportation Research Part A: Policy and Practice, Vol 162.
Near misses: C. Xu, J. Gao, F. Zuo, and K. Ozbay. (2024). Estimating Urban Traffic Safety and Analyzing Spatial Patterns through the Integration of City-Wide Near-Miss Data: A New York City Case Study. Applied Sciences, 14(14), 6378.
Collaborators
Collaborating with over 10 agencies, industry leaders, and academic partners, our work has been recognized in IEEE Spectrum and the U.S.DOT ITSJPO’s Deployment Case Study. Pilots with local agencies like the NYC Department of Design & Construction, U.S. DOT’s Connected Vehicle Pilot, and the Port Authority’s JFK Airport redevelopment project showcase our commitment to filling data gaps and enhancing traffic operation, asset management, event management, and emergency response in urban transportation systems.
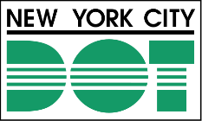
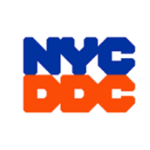



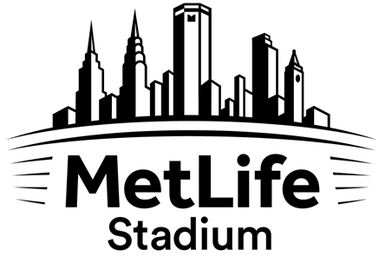


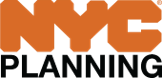
Use Cases
Urban Work Zone Detection and Sizing
This study developed a data-centric-based work zone object detection model with augmented multi-source training data (traffic cameras, web-mined images, and 3D simulations). It introduced innovative methods like topology-based inference and a reference-free size estimation to automatically recognize and size work zones in complex urban environments. The algorithms are integrated into a web-based tool that contains a dashboard and allows users to visualize real-time work zones on an interactive map.
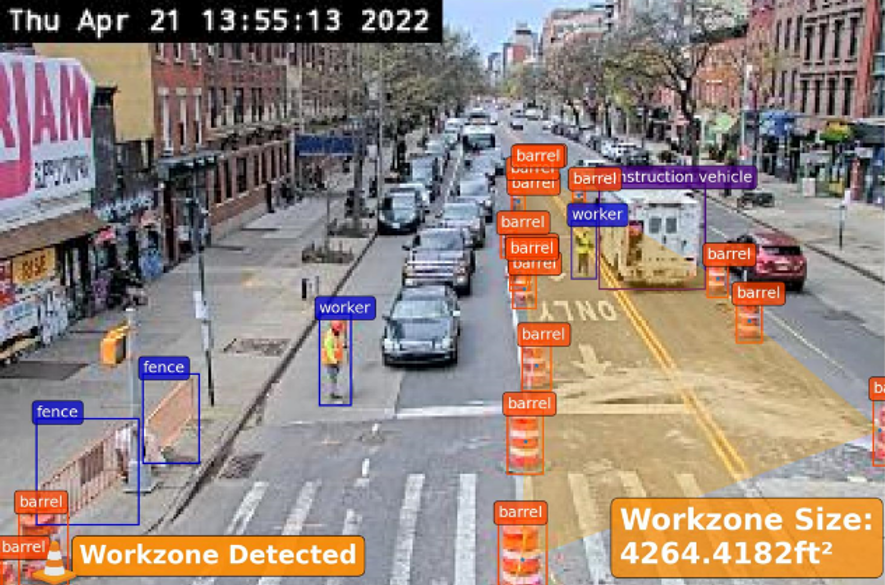
Bike Lane Pavement Distress and Street Hardware Detection
The research team piloted an innovative multi-sensor data acquisition system, the BSAFE-360, incorporating image recognition technology, mountable on bicycles, scooters, or dedicated vehicles—to systematically collect and analyze pavement condition data, especially for bike lanes. Partnering with NYCDOT, the pilot cataloged 13 types of pavement defects and street hardware (such as drains and manholes) through visual scanning and vibration sensing. This effort advances New York City’s ability to provide safe conditions for bike and scooter users and sets a model for other cities worldwide.
Airport Landside Traffic Management
To improve landside congestion and safety monitoring during JFK International Airport’s $19 billion expansion, the C2SMARTER team is partnering with the Port Authority of New York and New Jersey (PANYNJ) to develop a computer vision-based analytics tool. Leveraging existing cameras, this tool provides real-time traffic data to assist the decision-making process for terminal curbside operations and frontage modifications. By using JFK as a demonstration, this project seeks to enhance the efficiency of airport traffic monitoring, ultimately benefiting the regional economy and improving passenger satisfaction.
Curbside Management and Illegal Parking Detection
Computer vision-based video analytics can be employed to automatically monitor curbside activities in real time. By identifying parking and bus lane usage, parking violations (e.g., double parking), and congestion issues, the technology helps facilitate new strategies for curbside management, reduce traffic congestion caused by illegal parking, and improve overall urban mobility. The C2SMARTER team developed a new computer vision-based approach (“rank, detect, and quantify impacts”) and tested it using three case study sites in Midtown Manhattan.
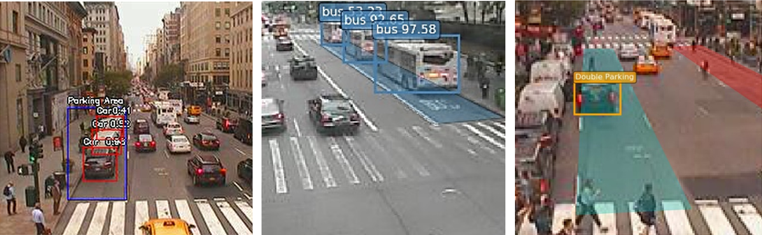
Near Miss Detection and Traffic Safety
Analyzing trajectory data extracted from traffic cameras, the C2SMARTER team was able to identify and analyze near miss incidents—situations where accidents are narrowly avoided—which are critical indicators of potential safety issues. By detecting unsafe behaviors and patterns involving vehicles, pedestrians, and cyclists, our application of computer vision to already available traffic camera footage can help cities implement proactive measures to improve road safety.
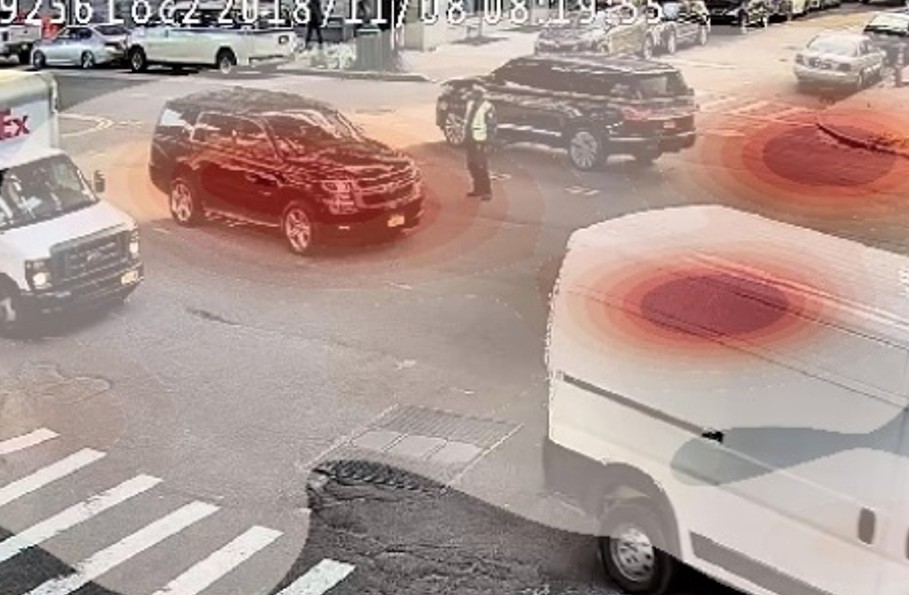
Traffic Monitoring and Performance Evaluation
Our computer vision-based solution for monitoring traffic flow and congestion in real time enables accurate measurement of traffic volumes, speeds, and patterns (e.g., speeding behaviors), providing valuable insights for traffic management and urban planning. Agencies can seamlessly integrate the technology with current traffic camera infrastructure to make data-driven decisions.
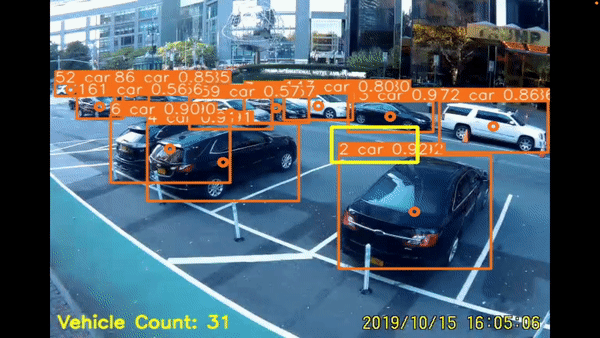
Pedestrian Detection and Improved Street Crossing for People with Disabilities
By applying advanced privacy-preserving image recognition algorithms, we can understand pedestrian movements in real time while maintaining individual privacy. Our framework identifies when pedestrians—including those with mobility challenges—are waiting to cross the street, enabling strategies such as adaptive traffic signals to provide safer and more accessible crossing opportunities.
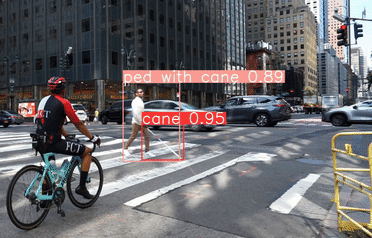
People
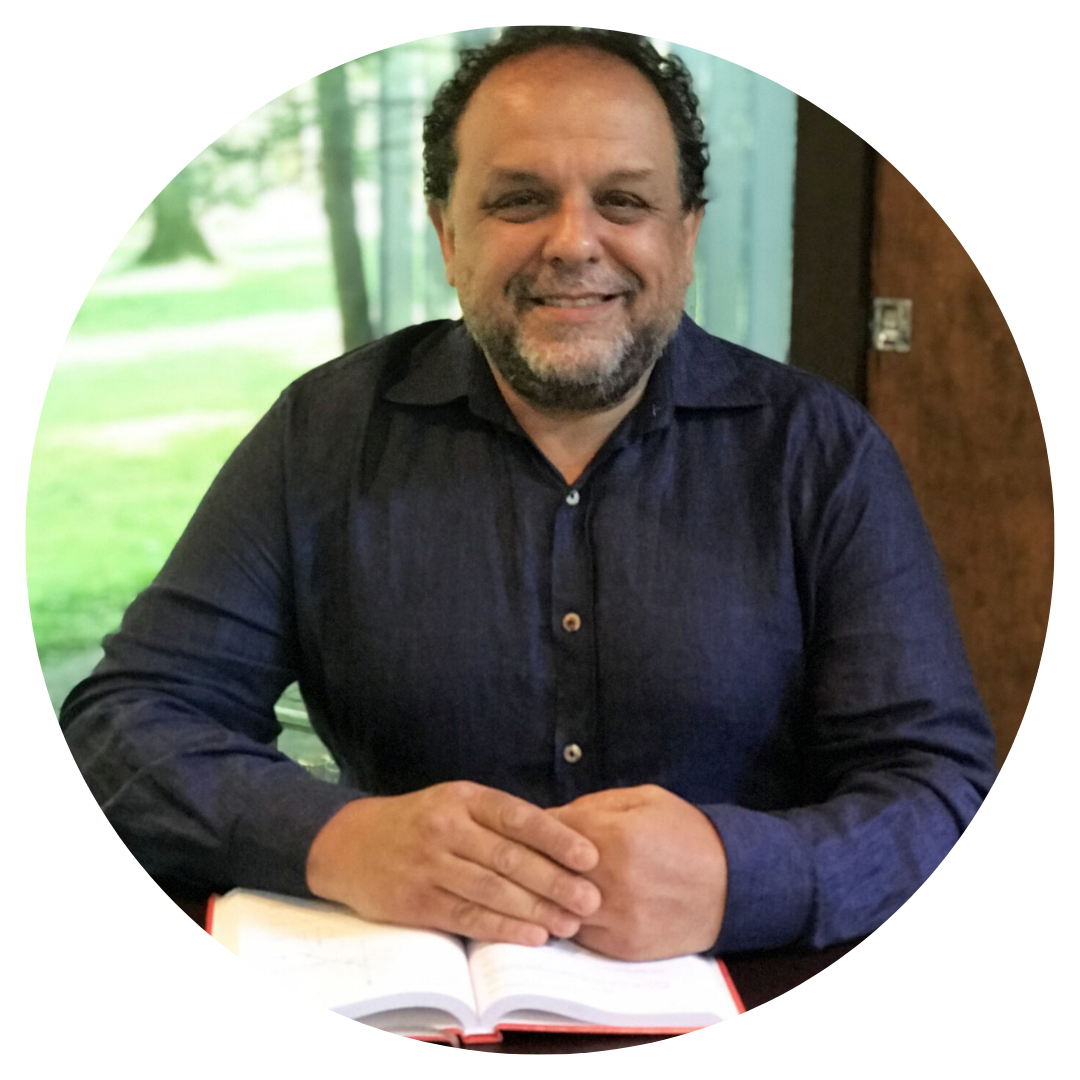
Kaan Ozbay
Director
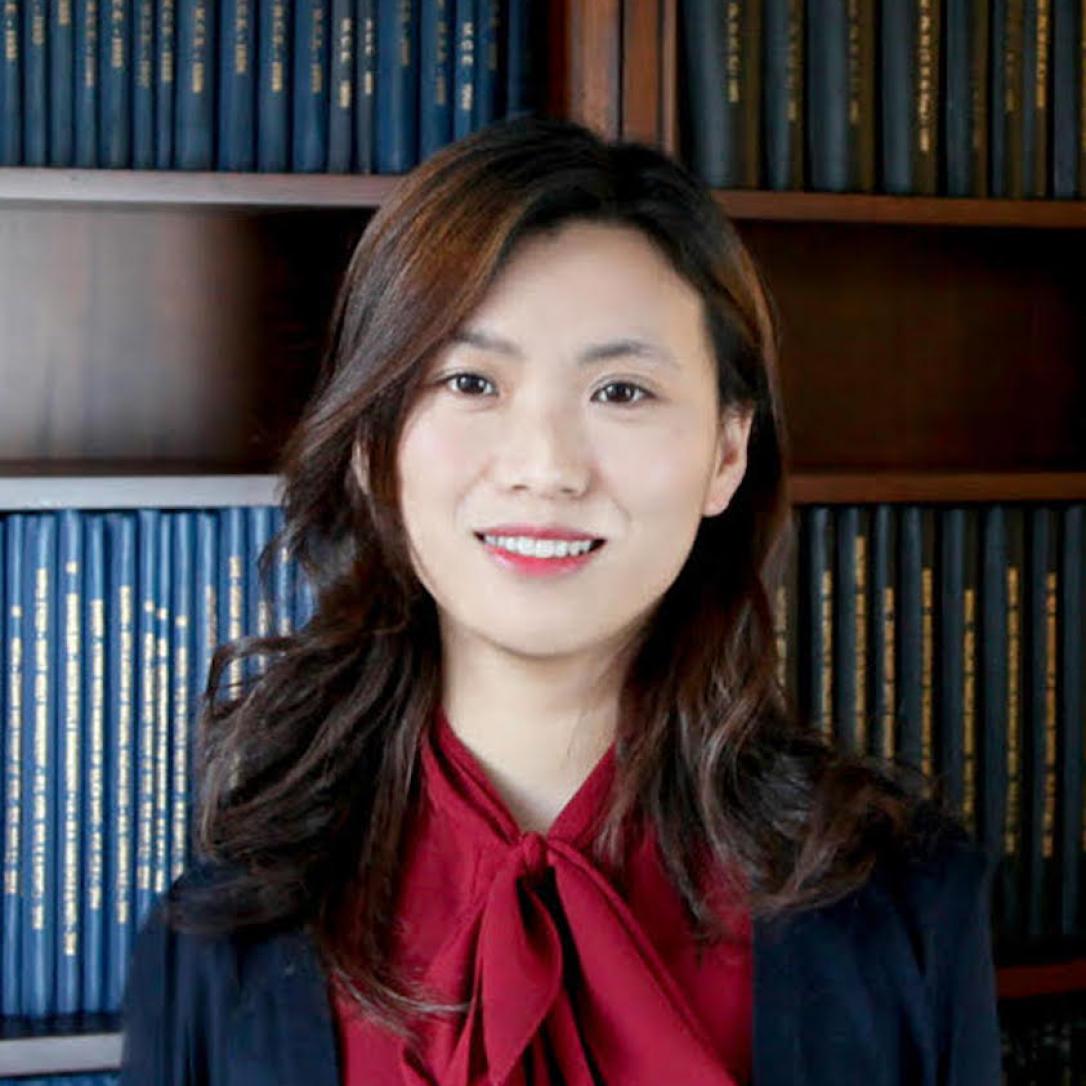
Jingqin Gao
Assistant Director
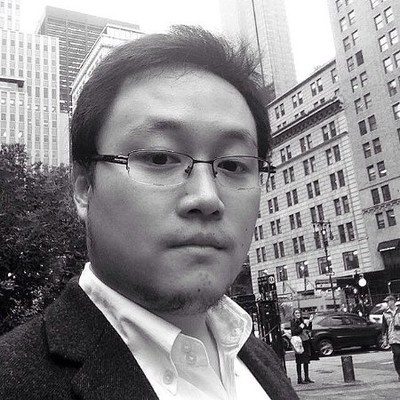
Fan Zuo
Post doctoral associate
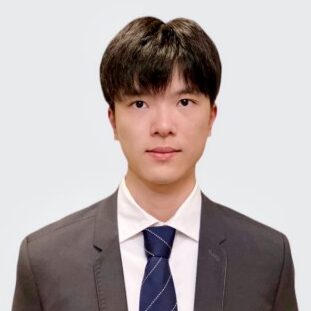
Donglin Zhou
Student Researcher