C2SMARTER is focusing on reducing congestion and ensuring that all our projects have long-term impact on the transportation systems in our Consortium cities.
Research Projects
C2SMARTER Projects
What is the New Baseline: Helping FDNY Understand Emergency Response Needs to Tackle Traffic and Service Congestion Under a Transient Population and Built Environment
PI: Joseph Chow
Co-PIs: Kaan Ozbay, Jingqin Gao
For Year 2, this ongoing partnership with FDNY will employ statistical and machine learning techniques combined with queueing-based optimization methodologies that relate American Community Survey (ACS) population attributes to high-severity calls and built-environment-affected response times, from which treatments for service bottlenecks can be prioritized. This project will also explore innovative EMS interventions, such as alternative vehicles, and provide deployment recommendations that could most benefit response times.
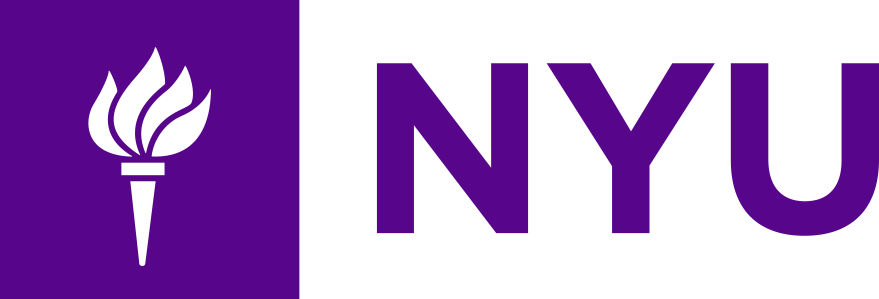
A Data-Driven Approach to Transportation Equity: Acquiring a Dataset of Heterogeneous Pedestrian Crossing Profiles
PI: Claudio Silva
Co-PIs: Jon Froehilch, Maryam Hosseini
The project’s goal is to collect a dataset of heterogeneous pedestrian crossing profiles in New York City. The team intends to do so by deploying high-resolution, high-frame-rate audiovisual sensors at intersections in the city.
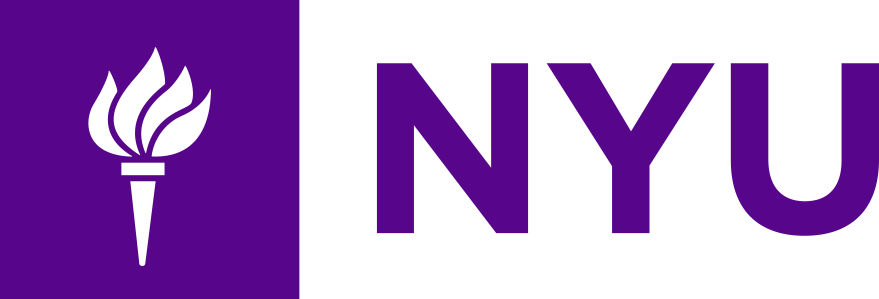
Enhancing Urban Micromobility Safety and Adoption through Biometrics and Mobile Sensing Technologies in El Paso, TX and New Brunswick, NJ
PI: Kelvin Cheu
Co-PI: Wenwen Zhang
This project will explore how street-level infrastructure design factors affect the perceived safety of e-scooters in El Paso, TX, and New Brunswick, NJ, using advanced biometric sensing technologies like eye-tracking glasses, galvanic skin response sensors, heart rate trackers, and video cameras. Researchers at UTEP and Rutgers will conduct e-scooter riding experiments with varied environments, infrastructure, demographics, and micromobility policies.
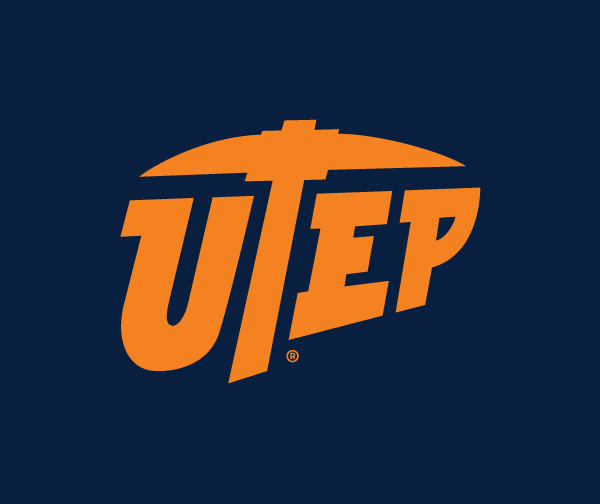
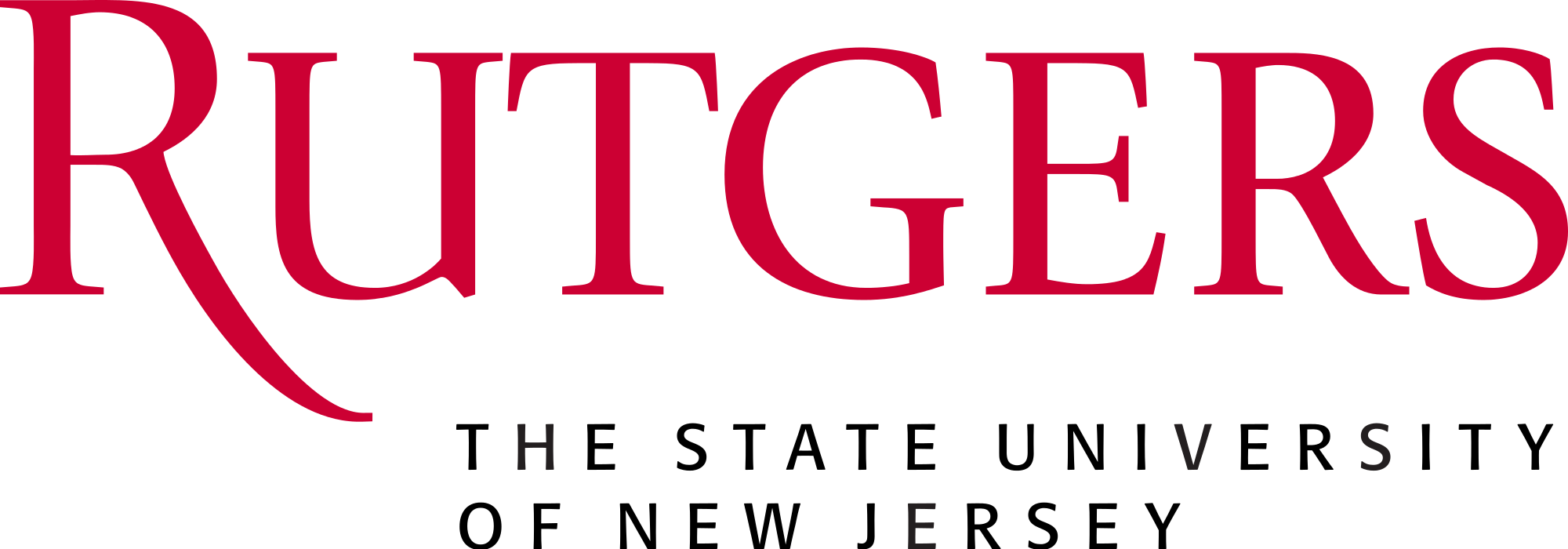
Red Light Camera Expansion in New York City: Spillover Effect, Behavioral Insights and Strategic Allocation
PI: Jingqin Gao
Co-PIs: Fan Zuo, Zilin Bian
This research project, in partnership with NYC DOT, aims to provide timely analytical support for the expansion of NYC’s Red Light Camera Program.
This research will help the city make data-driven decisions to maximize the program’s benefits throughout the expansion. The expanded network is expected to reduce intersection incidents, improve traffic flow, and decrease congestion, contributing to overall mobility and safety. The findings will also provide valuable insights for other states and communities with red light camera programs.
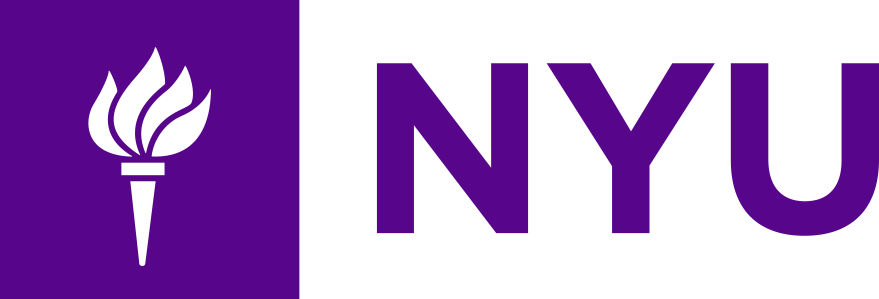
A Cost-Efficient Digital Twin Approach Using Pan-Tilt-Zoom Cameras to Enhance Urban Mobility Situational Awareness
PI: Zilin Bian
Co-PI: Kaan Ozbay, Fan Zuo, Wei Sun
This research will leverage the extensive, nationally deployed Pan-Tilt-Zoom (PTZ) camera network in a three-stage approach to enhance urban mobility situational awareness. The Urban Mobility Digital Twin (UMDT) will synthesize driver-centric information, detect safety and mobility risks, and support proactive decision-making in transportation management. Expected outcomes include enhanced insights and capabilities for states and communities using PTZ cameras.
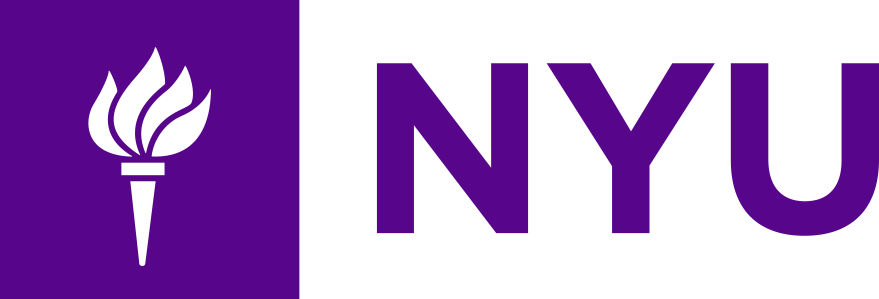
Amplifying Congestion Reduction by Integrating Macro and Local Traffic Control
PI: Jeff Ban
Co-PI: Angela Kitali
This project aims to develop optimal integration methods of macro-level perimeter control and micro-level traffic signal timing control to reduce congestion and improve multimodal mobility and safety. New methods, tools, and datasets will be developed, focusing on converting the optimal in/out flow from perimeter control to the actual control schemes of local traffic signals.
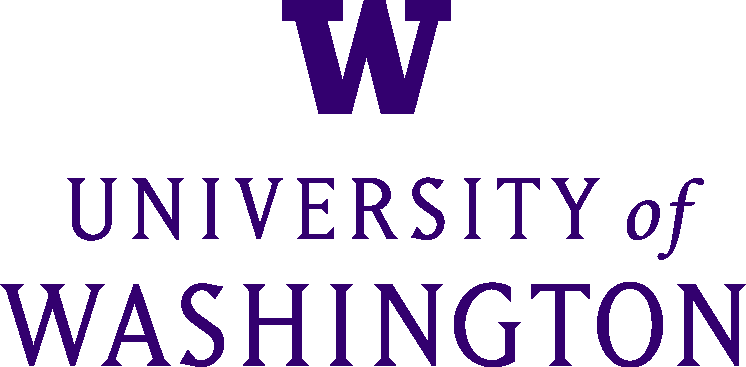
Improving Congestion Impact Estimation
PI: Eugene Vinitsky
The purpose of this project is to develop an accurate estimation of different congestion reduction schemes by creating more accurate digital twins. Current schemes are often benchmarked in micro-simulators but inaccurate micromodels of driver and pedestrian behavior can lead to the impacts being drastically over or under-estimated. The project goal is to improve these micro-models using new techniques in imitation learning.
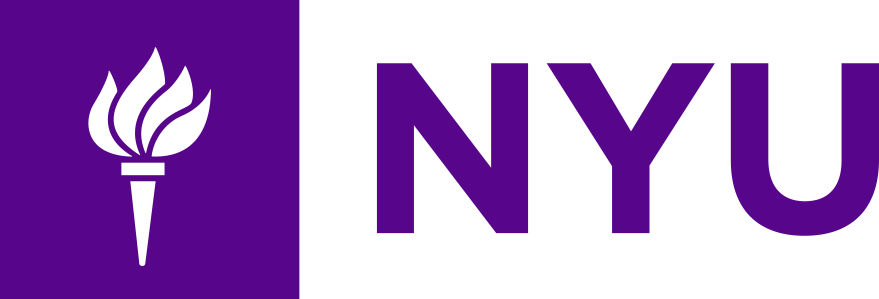
Equity Issues in Electronic Vehicle Adoption
PI: Yi Qi
Co-PI: Mehdi Azimi
This research proposal aims to investigate the equity challenges associated with EV adoption, focusing on socio-economic disparities, infrastructure accessibility, policy analysis, and potential solutions to foster more inclusive adoption.
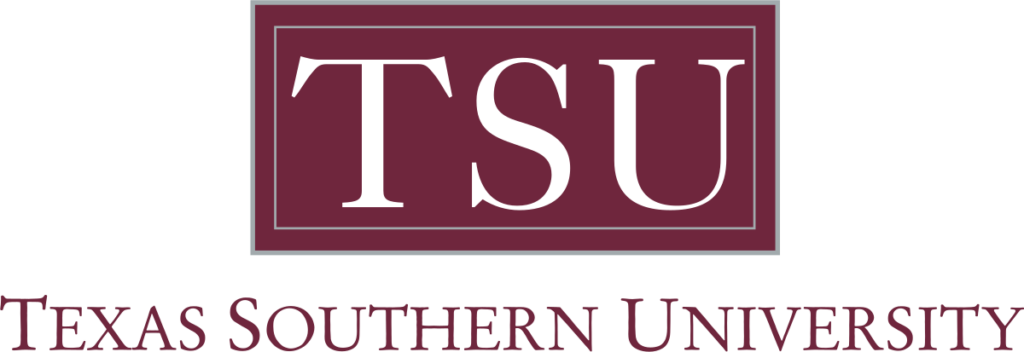
Digital Twins of Work Zones: Integrating Multi-Simulators to Quantify the Impact of Work Zones and Their Features on Traffic Congestion
PI: Semiha Ergan
Co-PI: Kaan Ozbay
This project intends to develop a virtual ecosystem with diverse agents, including vehicles, pedestrians, workers, and agents with disabilities, ultimately enhancing congestion reduction strategies and advancing simulation-driven planning.
The insights gained from this research will inform the design of more resilient transportation systems capable of adapting to evolving urban landscapes and mitigating congestion through data-driven strategies and innovative technologies.
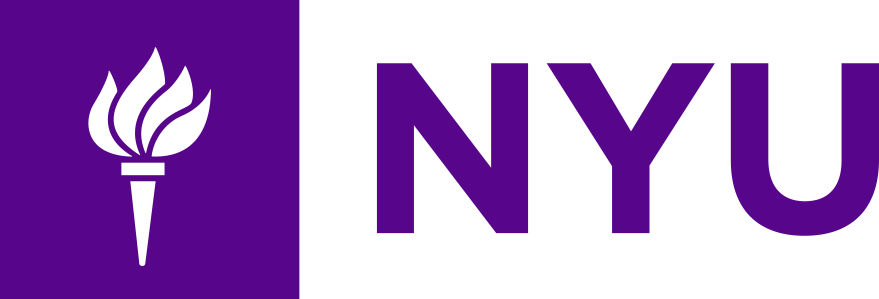
Understanding Travel Pattern Changes of Transit-reliant Population from Before to During and After Heat Wave: A Case Study for Seattle
PI: Cynthia Chen
This project will use ORCA data from King County Metro (KCM), the main transit company for the Seattle metropolitan area, to understand how travel patterns for the transit-reliant population changed during the 2023 heat wave in Seattle.
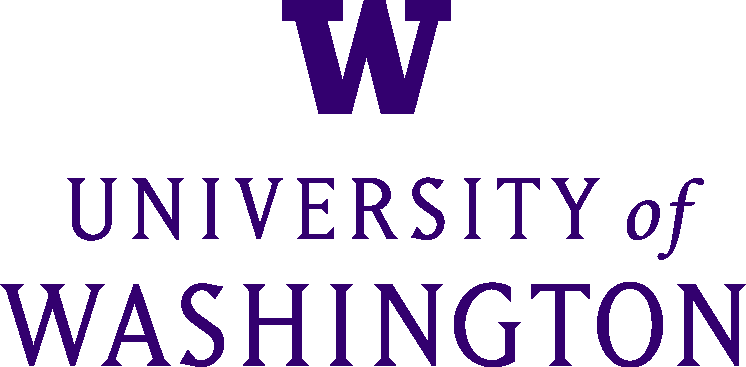
Real-Time Simulation Framework for Coupled Traffic and Power Grid Management During Disaster Scenarios
PI: Daniel Vignon
Co-PI: Chaoyue Zhao
This project will develop a framework and associated tools to address the challenge of real-time simulation of interconnected transportation and electric grid networks. This will enhance the preparedness of operators at both the city and regional levels during disaster scenarios, enabling them to manage congestion more effectively and ensure a coordinated response across both networks.
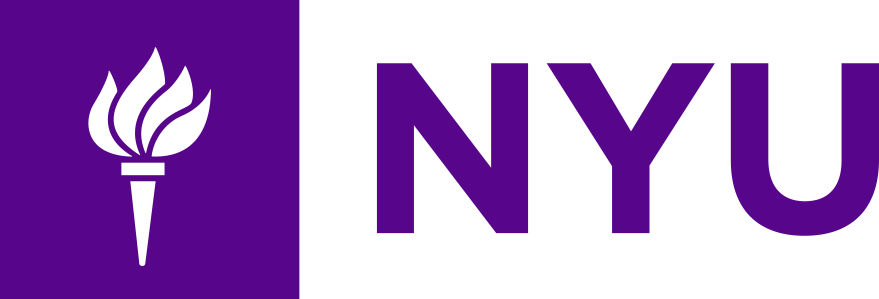
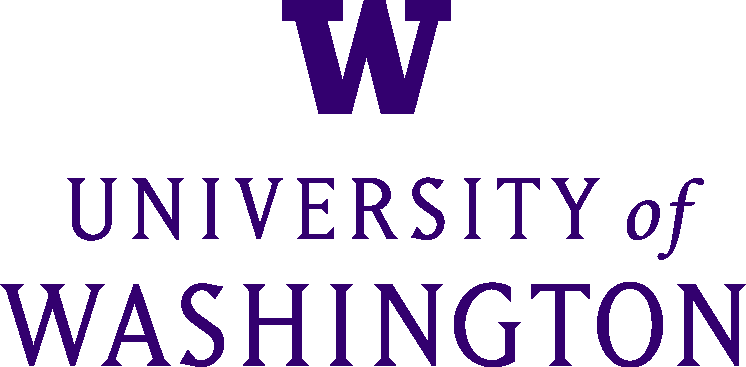
Assessment of Infrastructure Damage Cost and Compliance of Truck Weight Limit
PI: Hani Nassif
Co-PIs: Chaekuk Na, Wenwen Zhang, Patrick Lou, Kaan Ozbay
This project will synthesize a comparison between estimated damage infrastructure costs and violation fines associated with overweight trucks on the BQE corridor post-implementation of WIM-based direct enforcement.
The results will help guide legislative updates and support the creation of policies for setting appropriate violation fees through the use of WIM systems for direct overweight enforcement.
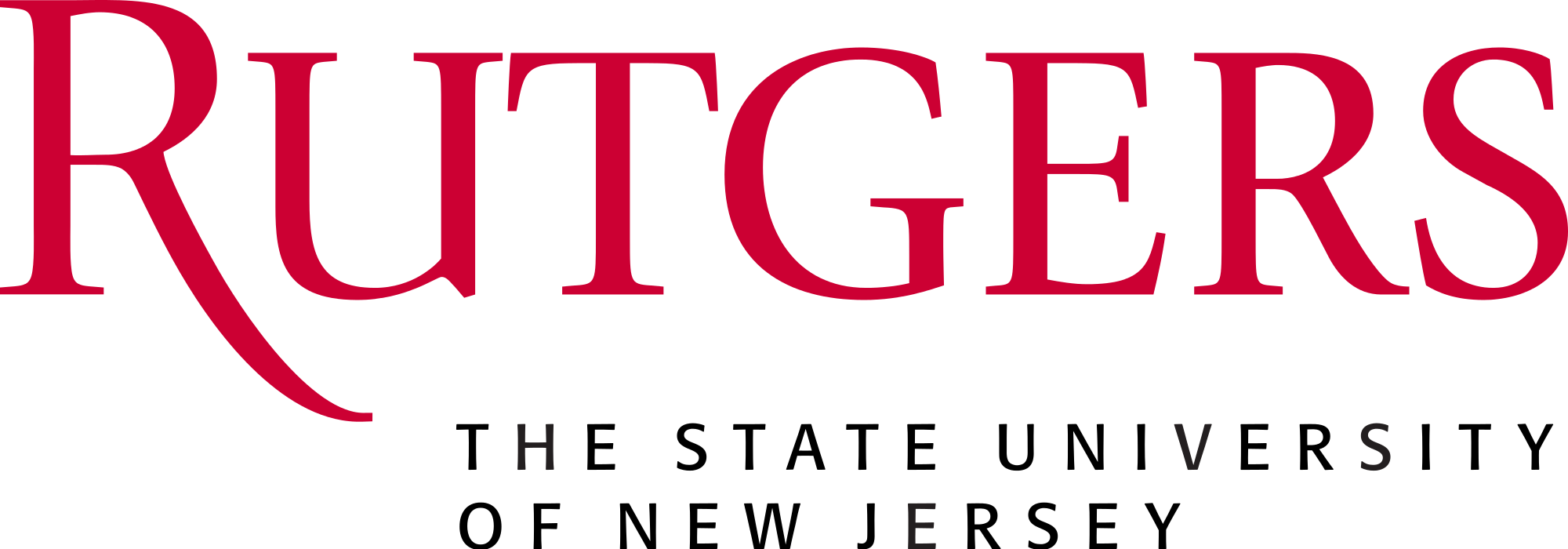
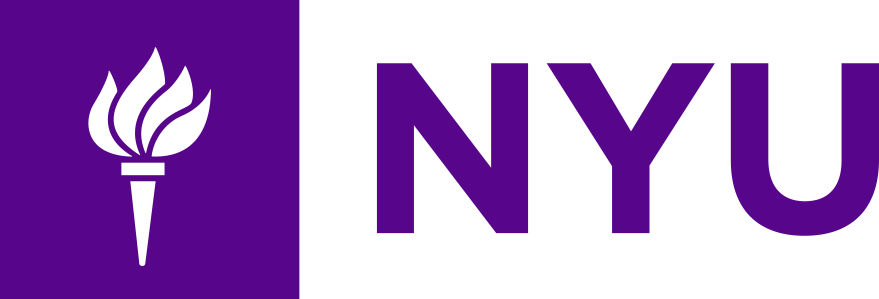
Integrating Personalized Incentives and Virtual Reality Tools for Enhanced Transportation System Management
PI:Venktesh Pandey
Co-PIs: Manoj Jha, Marwan Bikdash, Hyoshin Park, Shuva Chowdhury, Joe Chow
The proposed project employs a modeling approach to mitigate traffic congestion and enhance disaster management using personalized incentives and virtual reality (VR) tools. The main objectives include integrating personalized incentives with an existing multimodal trip planner, developing behavioral models to predict user responses, and using simulations to inform the design of incentive systems.
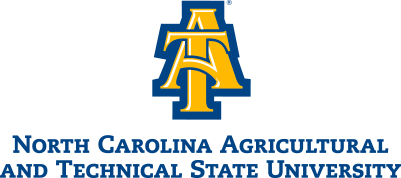
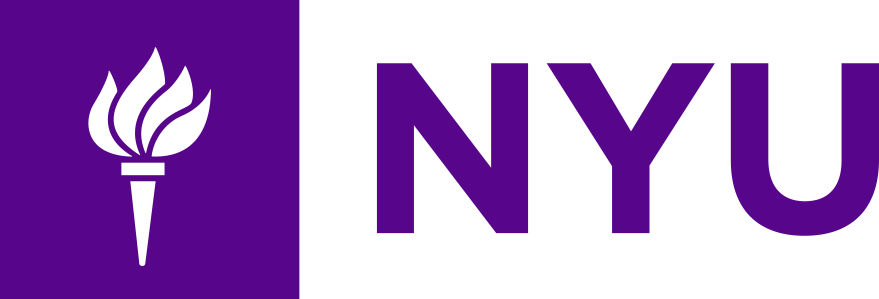
Estimating Daytime Population for Data-Driven Urban Planning
PI: Jingqin Gao
Co-PIs: Fan Zuo
Partnering with the New York City Department of City Planning, (NYC DCP), this project aims to leverage Artificial Intelligence (AI) and computer vision to analyze video data from over 900 traffic cameras across NYC to estimate daytime population.
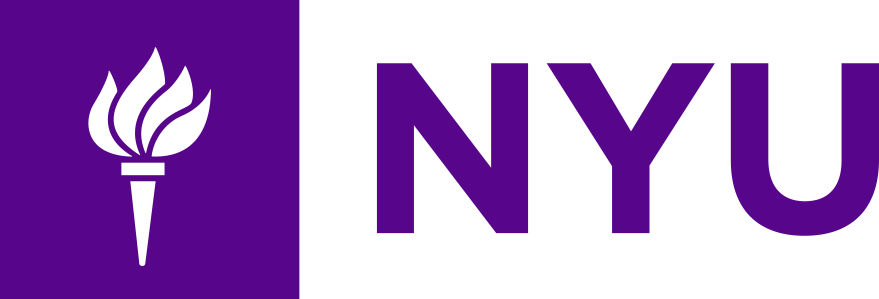
Physics-Informed Learning and Control of Connected and Autonomous Vehicles for Congestion Reduction
PI: Zhong-Ping Jiang
Co-PI: Kaan Ozbay
Building upon previous work, the goal of this project is to develop physics-informed machine learning and data-driven control-based tools for the combined longitudinal and lateral planning and control of connected and autonomous vehicles (CAVs).
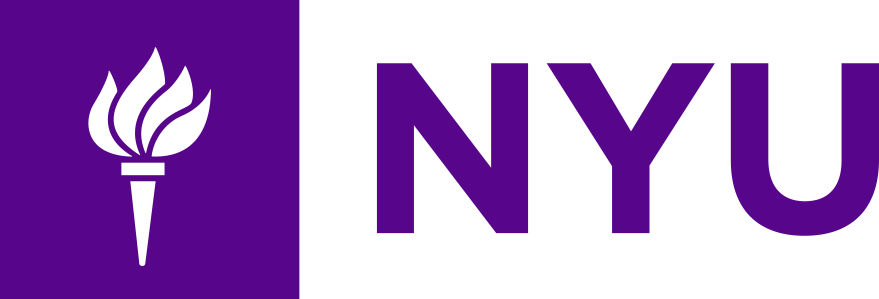
Education & Workforce Development
C2SMARTER Projects
Emerging Leaders in Transportation
PI: Sarah M. Kaufman
The Emerging Leaders in Transportation program is designed to give early-career, high-potential professionals the tools and connections to catalyze positive change in the transportation industry. It has run for eight programs over ten years. Through discussions, workshops, and activities, participants gain insights on paths to transportation leadership, identify career goals, and develop a lifelong network of industry peers.
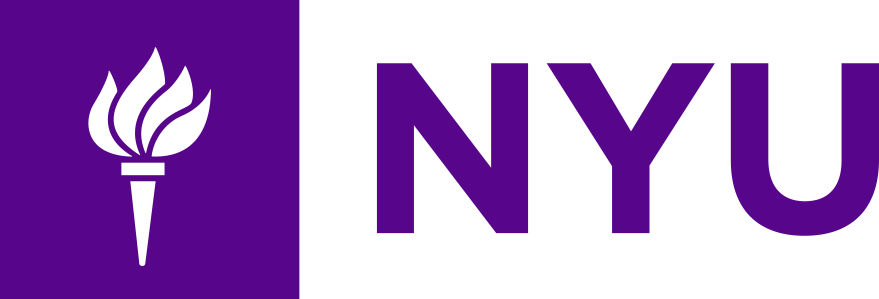
A Traffic Educational Hub: Learning by Deploying AI Models with Real Traffic Data
PI: Zilin Bian
Co-PIs: Kaan Ozbay
This project will develop an educational hub to facilitate the learning and application of artificial intelligence (AI) methods for transportation students. This hub will enhance students’ understanding of AI models by fostering their interest and willingness to experiment with real transportation datasets and deploy AI models in practical applications.
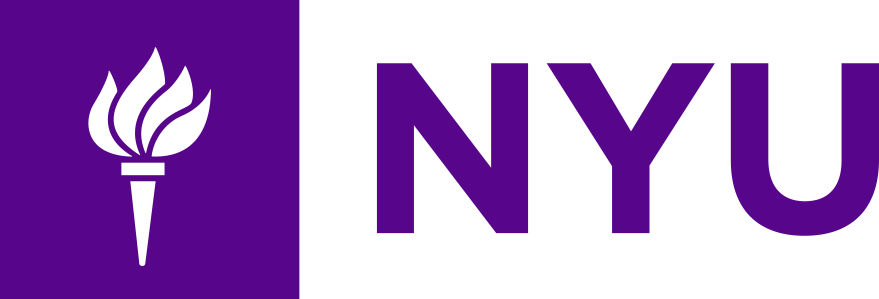
Workforce Development Pathways via Undergraduate Research
PI: Hamidreza Norouzi
Co-PI: Ivan Guzman
New York City College of Technology’s mission is “to provide broad access to high quality technological and professional education for a diverse urban population.” As the only College of Technology in the City University of New York, City Tech’s robust undergraduate research program serves over 400 student researchers annually. Research and engagement activities will focus on communities that are typically underserved and underrepresented in transportation equity.
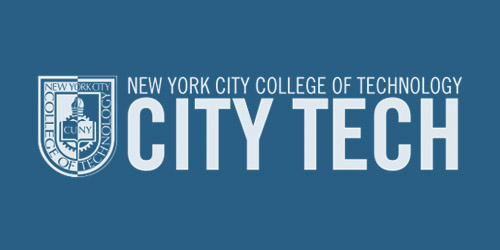
Technology Transfer Initiatives
C2SMARTER Projects
The Future of the Bus
PI: Sarah M. Kaufman
The Future of the Bus will consist of five stakeholder workshops, including bus, transit, and ride-hail experts; technologists and engineers; accessibility advocates; policymakers; real estate professionals; and energy experts from across the United States. It will also investigate the impact of autonomous technology on bus safety and its subsequent effect on reducing traffic congestion.
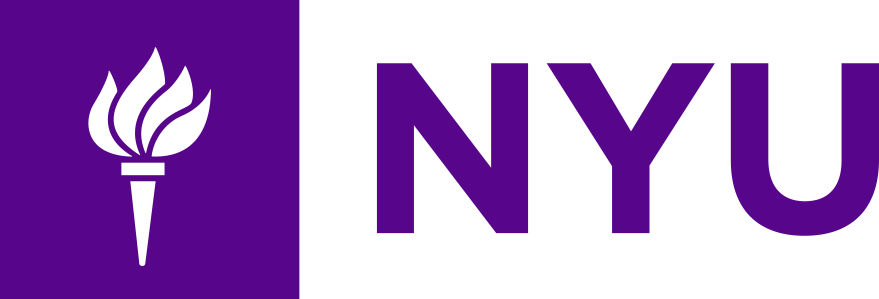