C2SMARTER 2023-24 Research Agenda
C2SMARTER is focusing on reducing congestion and ensuring that all our projects have long-term impact on the transportation systems in our Consortium cities.
Research Projects
C2SMARTER Projects
An AI-reinforced Traffic Digital Twin for Testing Emergency Vehicle Interventions
PI: Joseph Chow
Co-PIs: Kaan Ozbay, Jingqin Gao
Emergency vehicle (EMV) response times have degraded due to increasing urbanization and resulting congestion. Evaluating interventions to mitigate this degradation is too costly to be done in the field.This project will build a traffic digital twin (TDT) to be developed in collaboration with FDNY as a virtual test bed to evaluate interventions and support decision-making and planning in a safe simulation environment.The TDT will be built on the open source Simulation of Urban Mobility (SUMO) microscopic continuous traffic simulation.Key challenges are incorporating AI to learn non-EMV driver responses to EMV signals (sirens, V2X technologies) and to train the TDT to different traffic states using historical traffic data and dispatch data from FDNY.
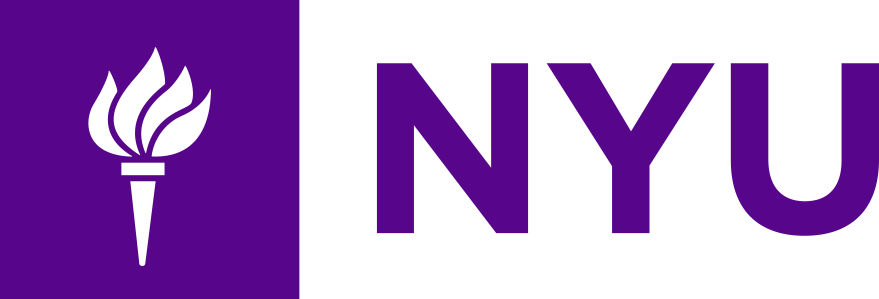
Equitable Flood Impact Analysis Integrating GeoAI and Digital Twin Modeling
PI:Venktesh Pandey
Co-PIs: Shuva Chowdhury, Hyoshin Park, Manoj Jha
This research proposal aims to explore the interdependencies between water and transportation infrastructures in urban areas. The flood risks and their impacts on infrastructure and livelihoods such as transportation mobility and accessibility will be assessed with a focus on social equity; for example, low-income households are more likely to live in high-risk zones, face higher damages, and are less able to recover. The project will use a data-driven approach to quantify the impacts at two scales: (1) macroscopic scale (such as a town or city) using GeoAI tools, and (2) microscopic scale (such as a census tract) using digital twin model. The research outcome will be useful in providing managerial and operational guidance on ways to address inequity issues during such events. The project aligns well with the USDOT’s sustainability goal by facilitating agency collaboration and addressing climate resilience.
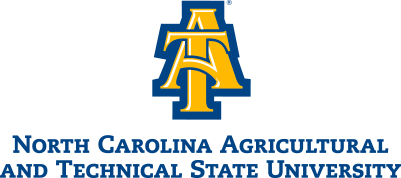
Enhancing Transit Access and Safety Through Equitable Micromobility Solution
PI: Kelvin Cheu
Co-PI: Adeeba Raheem
Micromobility refers to transportation enabled by small, low-speed, human- or electric-powered transportation devices, such as bicycles and scooters. Micromobility may be organized and deployed as a shared vehicle system, as the first and last-mile transportation mode to supplement transit. This project will investigate two major issues associated with the use of micromobility as a solution to improve the accessibility to transit in underserved communities. The first issue is related to the identification of micromobility stations in areas that are underserved by the fixed-route transit system. The second issue is the safety impacts of implementing micromobility in the abovementioned neighborhoods, and the related infrastructure improvements. A Concept of Operations (ConOps) of micromobility will be proposed to address the needs of the first and last-mile travel in consideration of traffic safety and infrastructure needs.
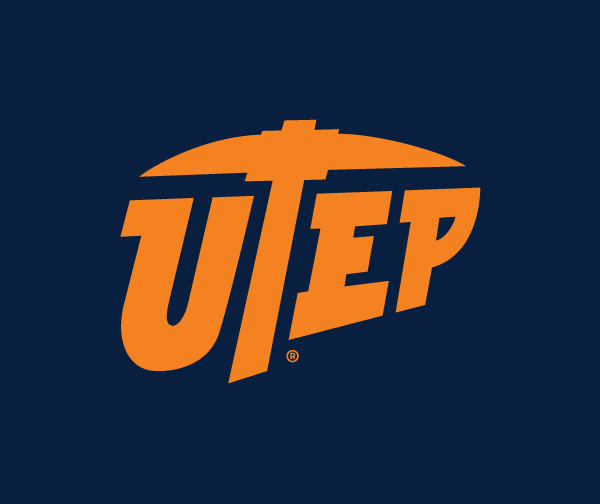
Multi-Horizon Urban EV Charging Infrastructure Planning: Integrating Activity Patterns, Grid Dynamics, and Uncertainty
PI: Daniel Vignon
Co-PIs: Jeff Ban, Chaoyue Zhao
While huge resources have been set aside for the deployment of Electric Vehicle (EV) charging infrastructure, optimally deploying EV charging stations to encourage the decarbonization of the transportation system is a non-trivial task. Indeed, EV charging interacts with several dimensions that operate on different temporal and spatial scales: activity patterns, electric grid operation, land use—to name a few. In this project, we aim to develop a multi-horizon planning model that can assist policymakers in determining the timing and location of EV charging stations in an urban environment. Our model incorporates several dimensions of decision-making, operation, and planning relevant to EV charging.
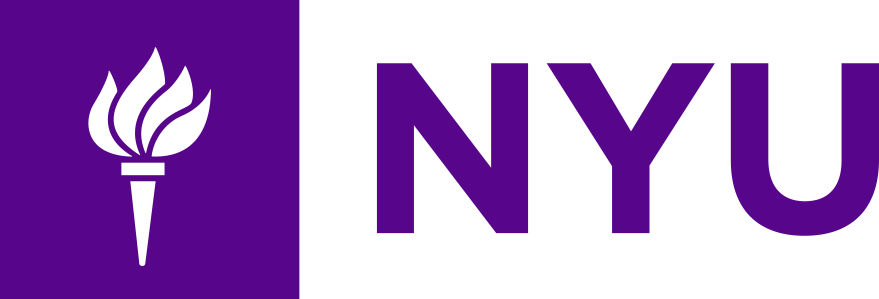
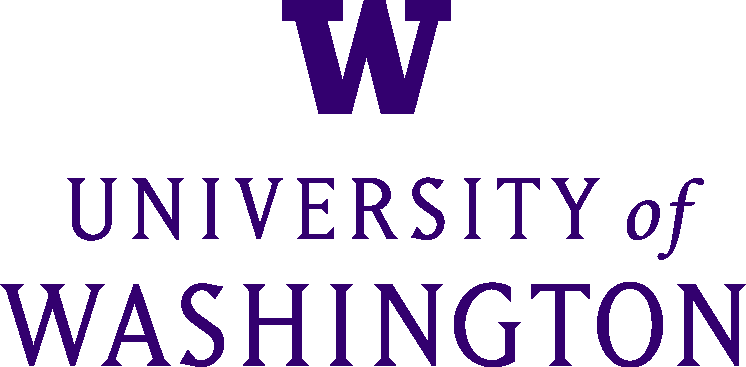
Development of a Vision-Based Landside Traffic Management System for Congestion and Safety Monitoring at Airports
PI: Jingqin Gao
Co-PI: Kaan Ozbay
Landside traffic management at airports is crucial for monitoring congestion and safety. Currently, The Port Authority of New York and New Jersey (PANYNJ) currently relies on traditional traffic data collection methods and manual observations from their cameras to monitor landside traffic at the John F. Kennedy (JFK) International Airport. This becomes especially significant given JFK’s ongoing $19 billion expansion and its daily service to nearly 62 million passengers. We propose developing an operationalized computer vision-based analytics tool in collaboration with PANYNJ that will leverage JFK’s existing cameras and provide real-time traffic data extraction, assisting the Transportation Management Center (PA-TMC) in its decision-making process. Key features include real-time traffic alerts, evaluations of the mobility and safety impacts from frontage modifications, and AI-driven assessments of construction zones. By using JFK as a demonstration, this initiative seeks to enhance the efficiency of airport traffic monitoring, ultimately benefiting the regional economy and improving passenger satisfaction.
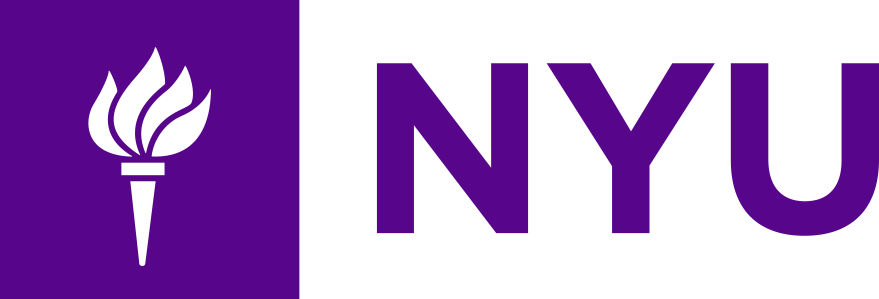
Multimodal Multiscale Urban Traffic Control in Connected and Automated Cities
PI: Jeff Ban
Co-PI: Angela Kitali
In phase 1 of this project, the team built a digital shadow of University of Texas – El Paso campus civil infrastructure and visualized impacts of the construction project schedule on the surrounding transportation infrastructure.
In phase 2, this project is focused on three aspects of expansion: (a) extending the digital twin (DT) model from a building to multiple representative locations on campus; (b) developing a Smart Living Lab (SLL), which enables live data streams from three selected sites on campus via sensor instrumentation, data communication, and database design; and (c) demonstrating a working prototype of digital twin, integrated with the digital model (DM) for real-time scenarios analysis, infrastructure interactions, and decision-making.
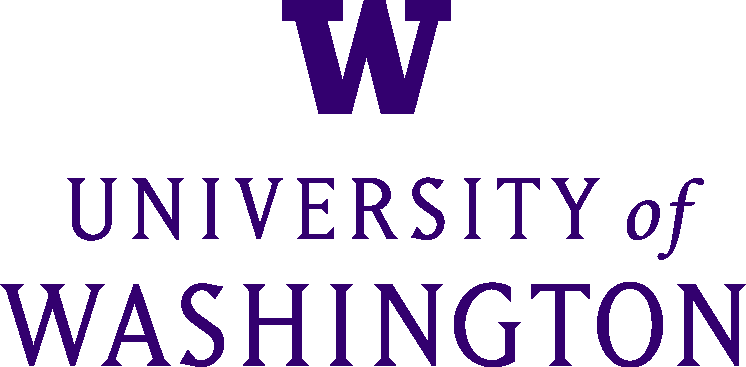
Building Intersection Digital Twins via GPU-Accelerated Human Regularized Reinforcement Learning
PI: Eugene Vinitsky
This work tackles a key limiting factor in the design of congestion reduction schemes, namely, the challenge of validating a proposed scheme with sufficiently realistic actor behavior. This is critical as oversimplified driving behavior models could artificially inflate or reduce the impact of a proposal. This project aims to tackle this challenge using new techniques in artificial intelligence and simulator design to enable the design of realistic driver models. A two-stage approach is proposed to design an efficient simulator to acquire the large number of samples needed to learn more complex models of human behavior at intersections. The team will useGoal-Conditioned Multi-Agent Reinforcement Learning (GC-MARL), combined with imitation learning, to build competent but diverse models of human behavior in dense traffic settings.
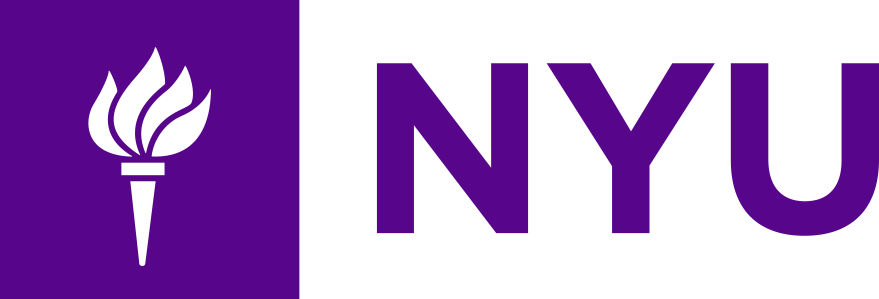
Development of Guidelines for Implementing the Flipped Left Diamond Interchange Design
PI: Yi Qi
Co-PI: Mehdi Azimi
This research is to investigate the operational performance of an innovative interchange design, Flipped Left Diamond Interchange (FLDI). FLDI can improve the capacity of the interchanges by minorly modifying of interchange geometric design. This modification does not require major construction and will overcome the problems in existing innovative interchange designs. However, because FLDI is a very new design, no study has been conducted to investigate the operational and design issues in the implementation. This study is to fill this gap and to promote and facilitate the implementation of this innovative design in the future. This research will also investigate the design issues in the implementation of the FLDI design and identify the applicable conditions for this new interchange design.
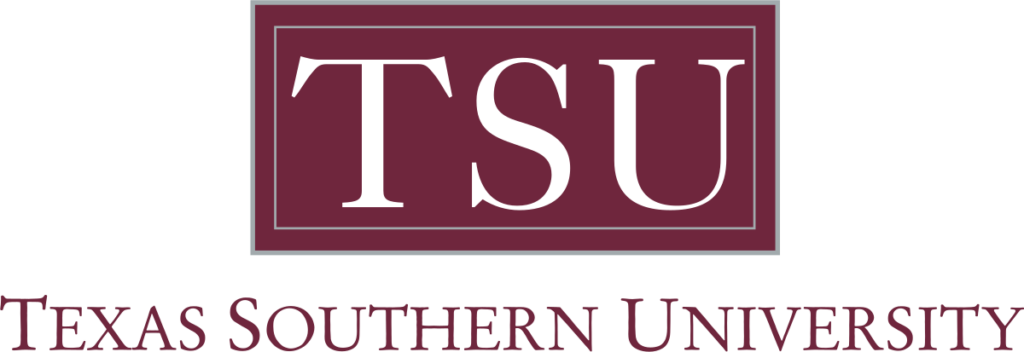
Sensor-enabled Calibration of VR-Integrated Co-Simulation Platforms for Enhanced Accuracy in Multi-modal Mobility Models
PI: Semiha Ergan
Co-PI: Kaan Ozbay
The proposed research project aims to embark on an innovative endeavor focused on the calibration of interactive and multi-simulation environments. Starting with SUMO and virtual reality environments, the project intends to create a vocabulary of calibration metrics for simulators, devise methods to expand data sources, and identify challenges on the way to establish a flexible framework for integrated calibration. Additionally, an existing testbed(e.g., Flatbush Avenue testbed)will be expanded to cover a disadvantaged community defined by USDOT Climate and Economic Justice Screening Tool in order to identify the challenges on the way to the proposed calibration platform and defining ways to measure its efficiency, accuracy, and robustness. This research strives to contribute significantly to the field of multi-modal mobility solutions, ultimately enhancing congestion reduction strategies and advancing the capabilities of simulation-driven planning.
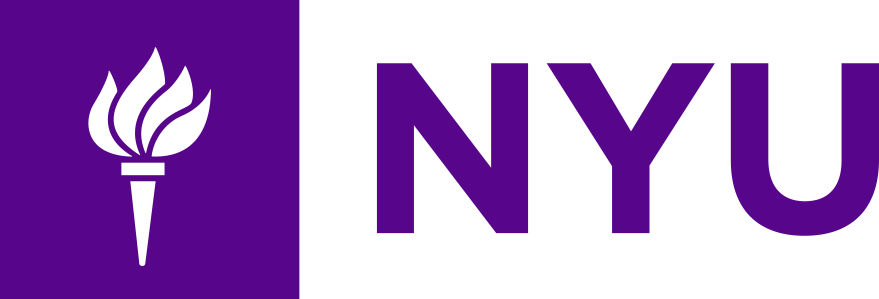
Impact of WIM-based Direct Enforcement on the Service Life of Bridges
PI: Hani Nassif
Co-PIs: Chaekuk Na, Wenwen Zhang, Patrick Lou
The Brooklyn-Queens Expressway (BQE) in New York City is a crucial corridor connecting the two boroughs of Brooklyn and Queens with other counties. Given its substantial daily traffic for transporting goods and services, the longevity of BQE is crucial to enhance public safety and alleviate congestion. The team has collaborated with NYCDOT to implement (1) an integrated weigh-in-motion (WIM) systems on the northern part of the BQE corridor for a direct enforcement of the high percentage of overweight (OW) trucks, and (2) a structural health monitoring (SHM) system to estimate the remaining service life of the BQE structures. This project synthesizes WIM and SHM data to study the impact of the reduction in OW percentage over time resulting from direct OW enforcement on extending the service life of the BQE. Furthermore, the team will conduct a life-cycle cost analysis (LCCA) of the network of bridges in NYC based on the established correlation. The output of this project will be a new framework to evaluate the effect of reduced OW percentage on the service life prediction.
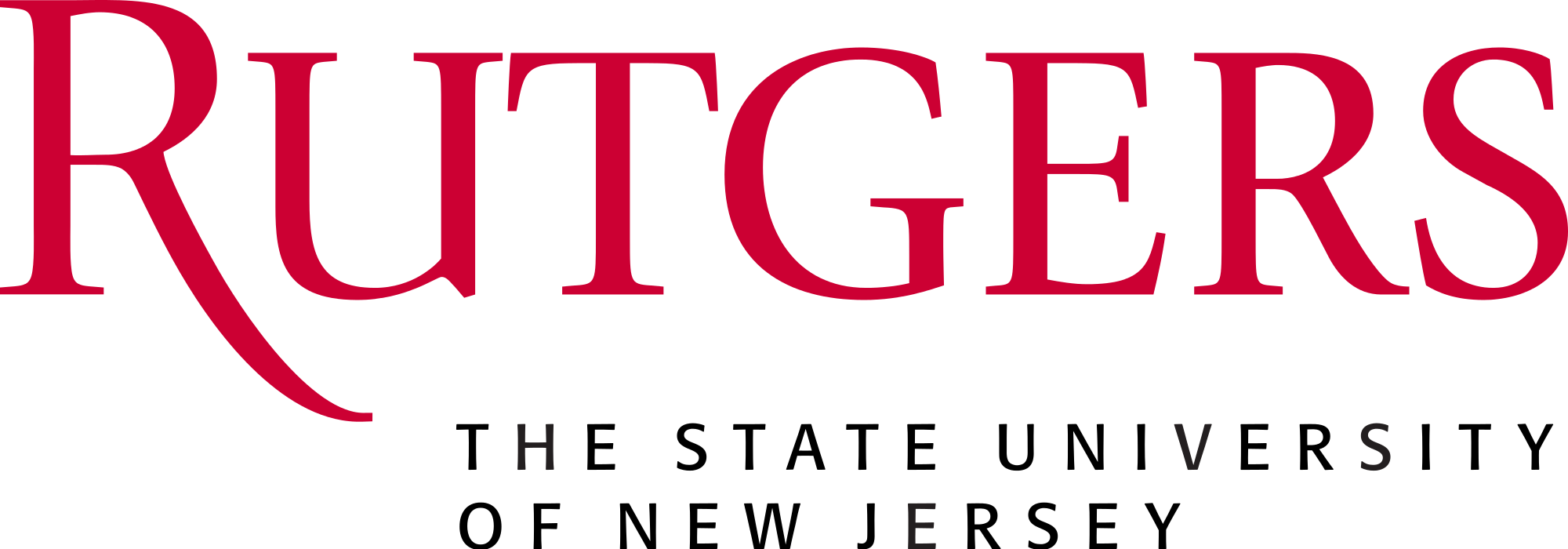
Multi-Modal Tripchain Planner for Disadvantaged Travelers to Incentivize Transit Usage
PI:Venktesh Pandey
Co-PIs: Joseph Chow, Hyoshin Park, Marwan Bikdash
This research project aims to enhance accessibility and mobility for disadvantaged travelers by developing an AI-powered multimodal tripchain planner. Current navigation solutions overlook the needs of people with mobility limitations, leading to challenges in accessing essential services. The proposed planner optimizes for multiple trips in a day, considering various modes and group-based travel preferences. Collaborating with partners like CoA, and Replica, the project tests the planner’s reliability and effectiveness on real-world instances. Outputs include an open-source tripchain planner, behavioral models for Arlington users, survey data demonstrating data collection capabilities, and revenue management strategy evaluation. This initiative promotes equity and reduces congestion by facilitating transit ridership, mobility for all users, and car use reduction. Ultimately, the project advances equitable mobility, supported by stakeholder collaboration and AI-driven analytics, in alignment with USDOT goals.
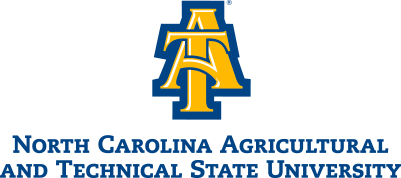
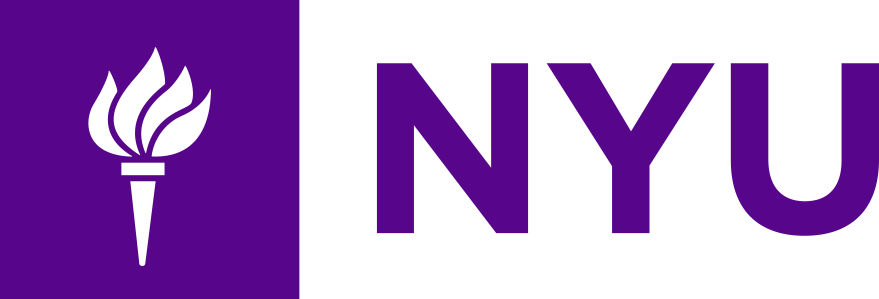
Control of Connected and Autonomous Vehicles for Congestion Reduction in Mixed Traffic: A Learning-Based Approach
PI: Zhong-Ping Jiang
Co-PI: Kaan Ozbay
Building on prior work on lane keeping and lane changing for connected and autonomous vehicles, this collaborative research project aims at taking a significant step forward to develop innovative learning-based, real-time control algorithms for connected and autonomous vehicles to reduce traffic congestion.This project aims at achieving four major objectives: 1)developing a traffic light prediction method by utilizing advanced deep learning techniques; 2) developing a trajectory optimization framework fora stream of vehicles to efficiently reduce the traffic congestion, attenuate the stop-and-go waves, and increase the throughput of the traffic; 3) integrating reinforcement learning techniques with (control) barrier functions to address the safety-oriented learning-based trajectory tracking control of autonomous vehicles; 4) validating the proposed congestion-reducing scheme with real-world vehicle trajectory data and SUMO testing under different environments in the presence of different vehicle mixes and driver uncertainties.
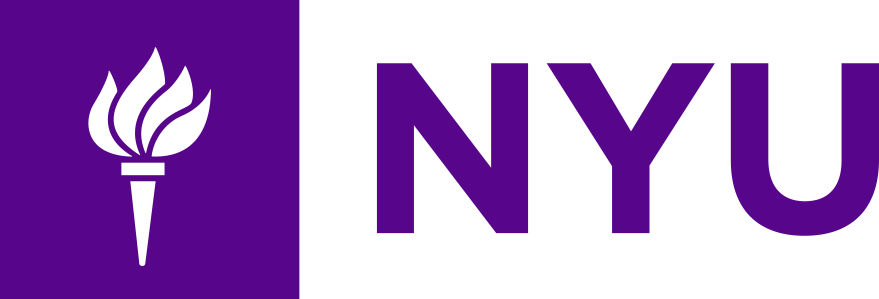
Education & Workforce Development
C2SMARTER Projects
K-12 STEAM Club Drone Tech for Hazards Resilience and Transportation Workforce Development in Rural, Isolated, Tribal and Indigenous Communities
PI: Daniel B. Abramson
Co-PI: Jeff Ban
This project builds on a relationship and program established at the Ocosta Junior High School After School Program and the City of Westport in South Beach, WA, under a series of Center for Safety Equity in Transportation (CSET) projects in Rural, Isolated, Tribal and Indigenous Communities since 2018. This grant’s contribution would provide essential support to local school staff to coordinate with the University of Washington and the City of Westport for activities that involve youth in research and training on the above topics, and to conduct outreach and recruiting for senior high school students and Tribal youth (who attend the Ocosta Schools) to participate and obtain their FAAPart 107 drone pilot licenses. Students participating in the program will learn to apply drone video and video editing skills to citizen science initiatives in a community that is existentially threatened by a Cascadia Subduction Earthquake and Tsunami as well as climate change-driven sea level rise and worsening coastal floods, landslides, and erosion.
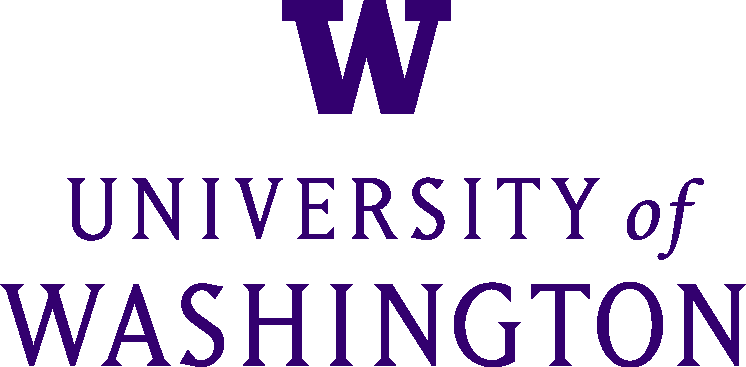
Emerging Leaders in Transportation
PI: Sarah M. Kaufman
The Emerging Leaders in Transportation program engages early-career transportation professionals to develop and promote innovations within their organizations to improve mobility practices in the New York region. Participants develop plans to incorporate innovative tools or practices within their organizations, such as new workflows, data analysis tools, networking activities and access to educational programs. The program has successfully completed seven Emerging Leaders programs, with nearly 150 participants since 2014.Participants report high satisfaction rates with their knowledge exchange and leadership development. They also appreciate time with executives, industry leaders, as well as one another, and maintain these contacts throughout the years. Transportation agencies and companies enjoy new efficiencies and programs, as well as employees who are more ready to take on leadership roles.
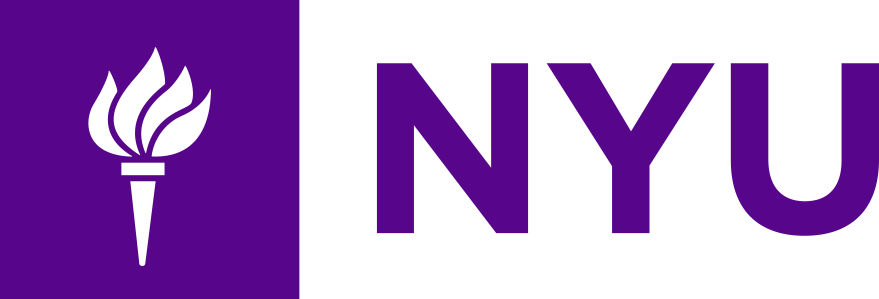
Tech Transfer with Undergraduate Research & Workforce Development
PI: Gerarda M. Shields
Co-PI: Hamidreza Norouzi
New York City College of Technology’s mission is “to provide broad access to high quality technological and professional education for a diverse urban population.” Over 85% of our students are native New Yorkers who, as graduates, will support the local economy. For over 25 years City Tech has been designated as a Hispanic Serving Institution, forwarding accessible education and training. As the only College of Technology in the City University of New York, City Tech’s robust undergraduate research program serves over 400 student researchers annually. Research and engagement activities will focus on communities that are typically underserved and underrepresented in transportation equity.
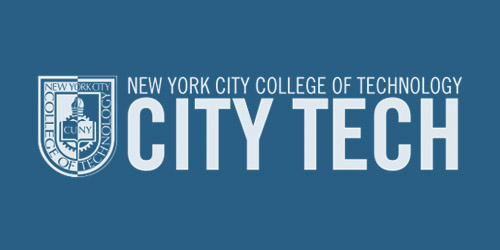
Technology Transfer Initiatives
C2SMARTER Projects
Charting Equitable Outcomes for Highway Communities/Smart Technology and Smart Development
PI: Marc Norman
Co-PI: Matthew Kwantinetz
There is now a growing body of evidence and precedents for repairing the harm done to U.S. communities due to the destructive paths and policies that create urban highways. We have seen the possibilities of creating better climate outcomes (local and global), reduced congestion, greater economic development potential and enhanced cultural assets. Cities such as Seoul, San Francisco, Milwaukee, and Seattle which have successfully reimagined highways for more equitable and connected outcomes will be brought in dialog with those in the planning and design stages such as Detroit, Syracuse, Portland, Rochester and New York City. Embedded in the positive change is the use of technologies for controlling and redirecting traffic flows and state of the art thinking in the ways congestion and induced demand affect larger urban systems. Researchers in a range of fields will come together to discuss successes and create best practices for the coming wave of urban highway rethinking. NYU’s Schack Institute of Real Estate will partner with NYU Tandon and University researchers at colleges, institutes and labs in cities at the forefront of equitable highway transformation.
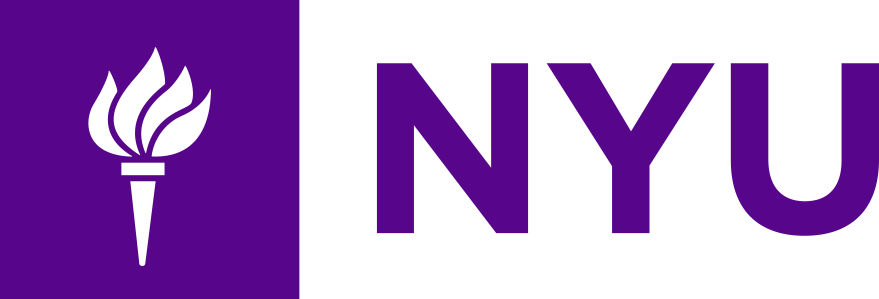
Transit Accessibility Showcase
PI: Sarah M. Kaufman
The Transit Accessibility Showcase will demonstrate new and emerging technologies to assist people with disabilities in using public transportation.
The program–a panel discussion and technology showcase-will generate new innovations and connections among policymakers, advocates and riders to make public transportation more usable by all.
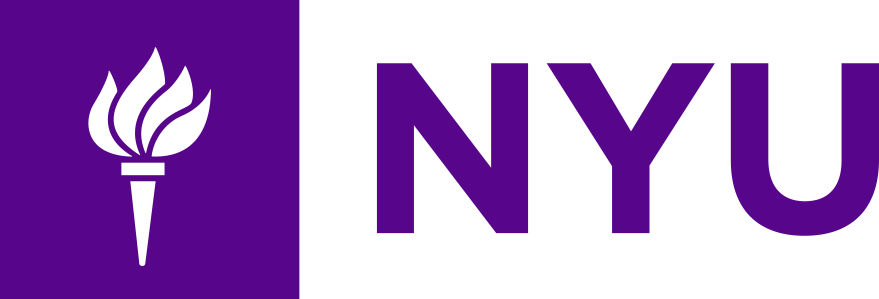
A Knowledge Capture and Deployment Tracking Platform for UTC Research
PI: Jingqin Gao
Co-PIs: Fan Zuo, Zilin Bian
This research will develop a knowledge base to track the deployment of ITS projects and summarize their equity outcomes, performance measures, tools, benefits, costs, and best practices in contributing to US DOT strategic goals. The team will leverage its experience on a similar national ITSJPO project to collect, synthesize, and transfer knowledge. A web portal will be created to connect academic research and the state of the practice. Phase I of the project will start from University Transportation Center (UTC) research, especially on congestion reduction and studies that contain equity considerations in transportation. This knowledge base will especially focus on knowledge that has not been studied well in the past practices, including categorization of new measures of effectiveness (MOE) used for emerging technologies, summary of tech transfer methodology, operationalized tools and outcome of the research, as well as equity aspect of the deployments. AI-based solutions, including large language models (LLMs), will be developed to assist the knowledge synthesis process with manual validation to evaluate the effectiveness of using this AI-based approach.
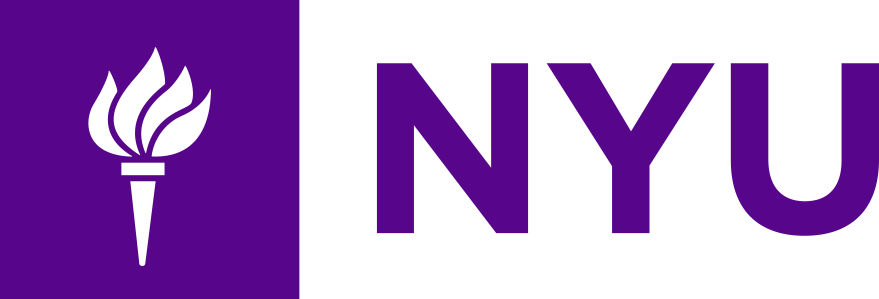