C2SMART 2022 Research Agenda
C2SMART is focusing on collaboration, implementation, and equity, looking to ensure that all our projects have long-term impact on the transportation systems in our Consortium cities.
NY Statewide Behavioral Equity Impact Decision Support Tool with Replica
PI: Joseph Chow
In partnership with Replica, the research team will build out a behavioral response decision support tool designed to measure impacts on equity for different transportation systems design change. A novel methodology based on inverse optimization will be used so that deterministic heterogeneous parameters can be derived for each individual to allow for more consistent, interpretative behavioral response modeling for large-scale maximization of equity in service region design.
This study will also identify under-served communities in rural areas that would best benefit from introducing new mobility services under a fixed statewide deployment budget.
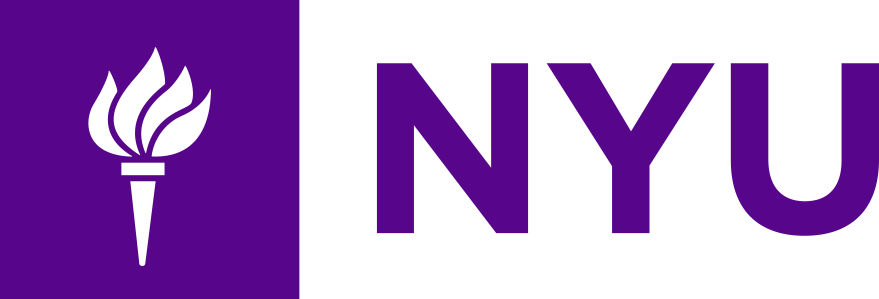

One-to-Many Simulator Interface with Virtual Test Bed for Equitable Tech Transfer
PI: Joseph Chow
Co-PIs: Kaan Ozbay, Jeff Ban
Building on five years of R&D with MATSim-NYC, the research team will develop a broad API to interface any simulation with a multi-agent demand simulator. This will be tested on the existing MATSim – NYC (which will be enhanced to include freight and parcel delivery activities) and a BEAM implementation, BEAM – NYC, for three use cases in electric transit, freight, and traffic, considering equity impacts on different population segments (by income level, having disabilities, age level).
This research will take place jointly in NYC and Seattle, enabling deeper insights of evaluated cases and promote tech transfer and collaborations to broader communities (including agencies, the industry, and the public).
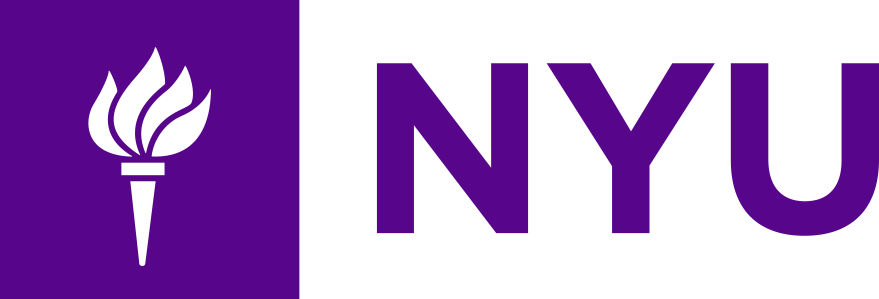
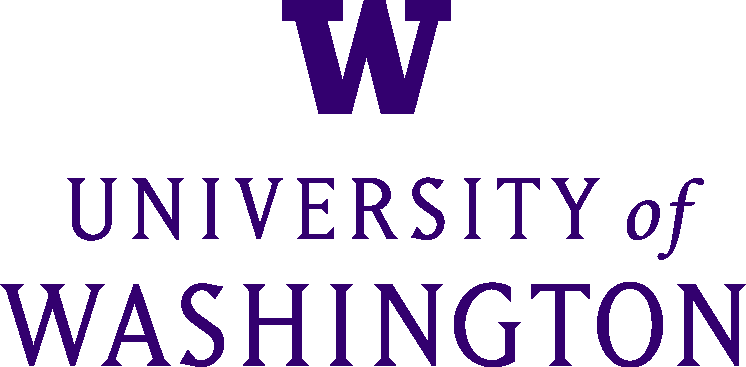

An Artificial intelligence (AI) Based Overheight Vehicle Warning System for Bridges
PI: Anil Agrawal
Impact of overheight vehicles on bridges is a major problem that causes extensive damage to bridges and leads to frequent traffic and mobility issues. This research targets a very low – cost Artificial intelligence ( AI ) overheight vehicle warning system for bridges based on the use of cutting-edge camera technology, augmented reality and AI based height detection approach.
The proposed system could be installed on a bridge in the direction facing the traffic and could detect an incoming overheight truck from the safe stopping distance using the combination of image processing, augmented reality, and AI. Successful completion of the research would lead to the commercial development of a system that could be installed cost – effectively to prevent impacts to bridges by trucks nationwide.


Urban-DEPOT: Deploying EV Charging Infrastructure in an Urban Environment
PI: Yury Dvorkin
This project develops tools for forecasting electric vehicle charging demand and for placing and siting electric vehicles charging stations in an urban environment, while considering transportation, electric grid, and urban-space constraints.
The project engages two private-sector organizations which are directly associated with the proposed effort, Consolidated Edison and Charge Point, to facilitate real-world implementation, including development of a deployment decision support tool to help guide expanding electrification and charging station rollouts across New York City.
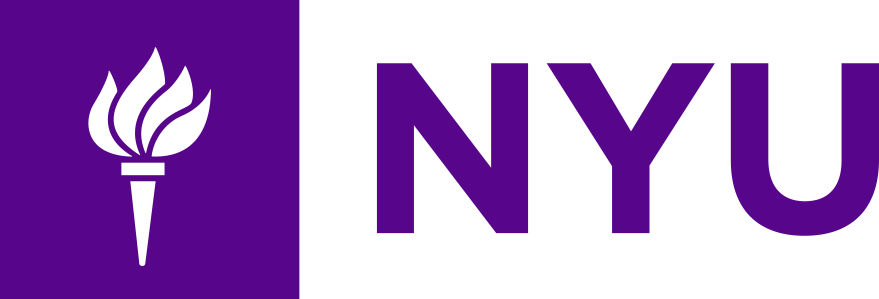
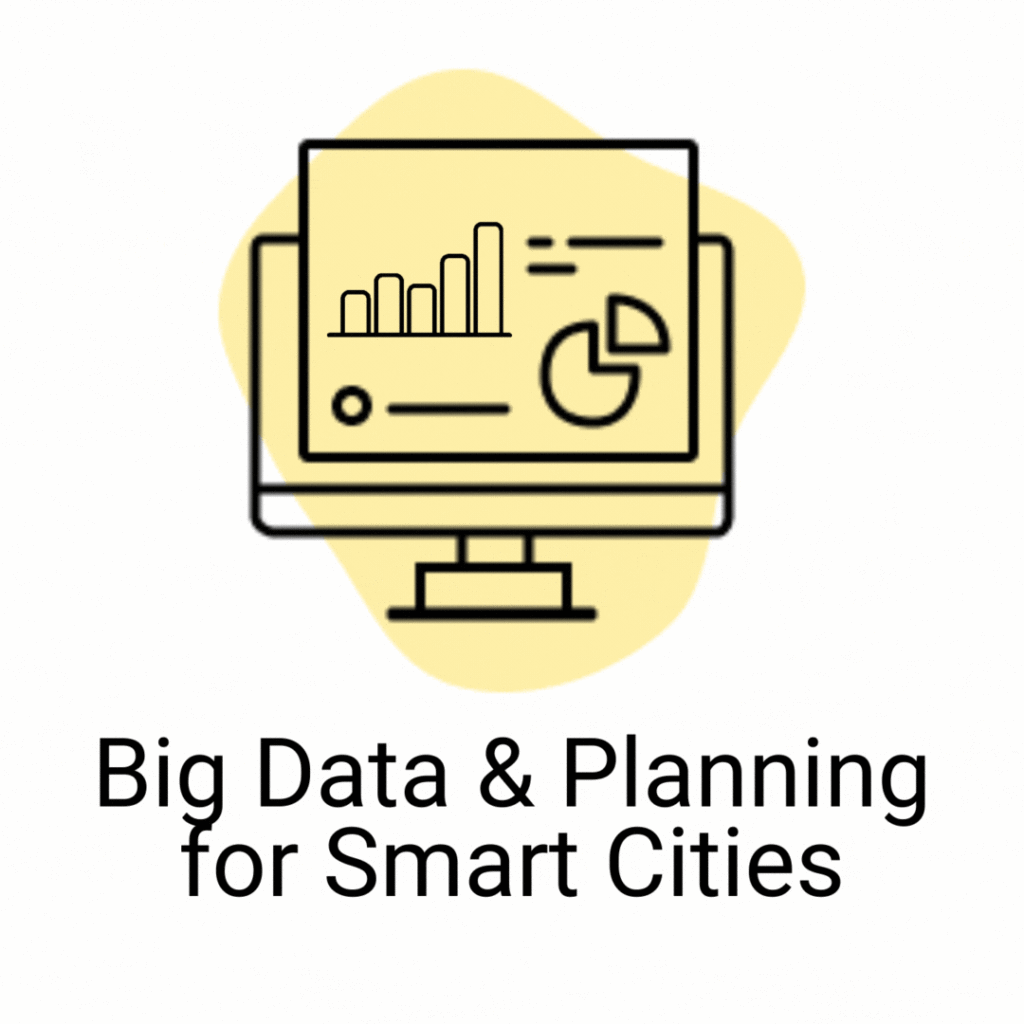
A Cost-effective Approach Towards Building a Traffic Sign Data Inventory Using Open Street Images
PI: Yinhai Wang
Traffic signs are critical assets for roadway and infrastructure management. They are also in a great variety and different conditions. The research team will propose a cost-effective approach to building a traffic sign data inventory using open street images.
The project consists of three primary goals: (a) a data collection system which can capture the real-time open street image data along with the associated information; (b) a traffic sign detection and recognition model to extract the information from original images; and (c) a data storage system which will store the recognition results as well as associate information into the database.
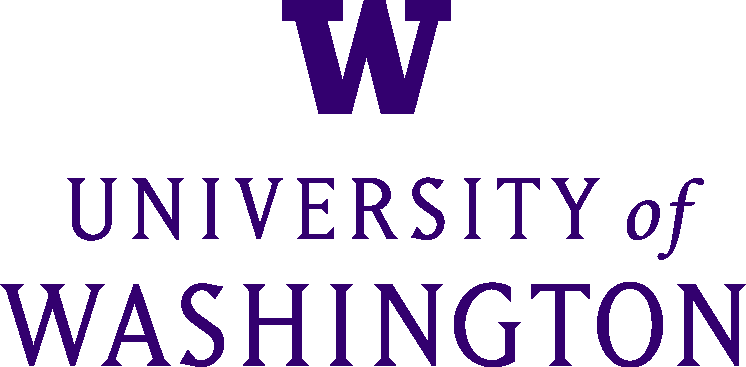
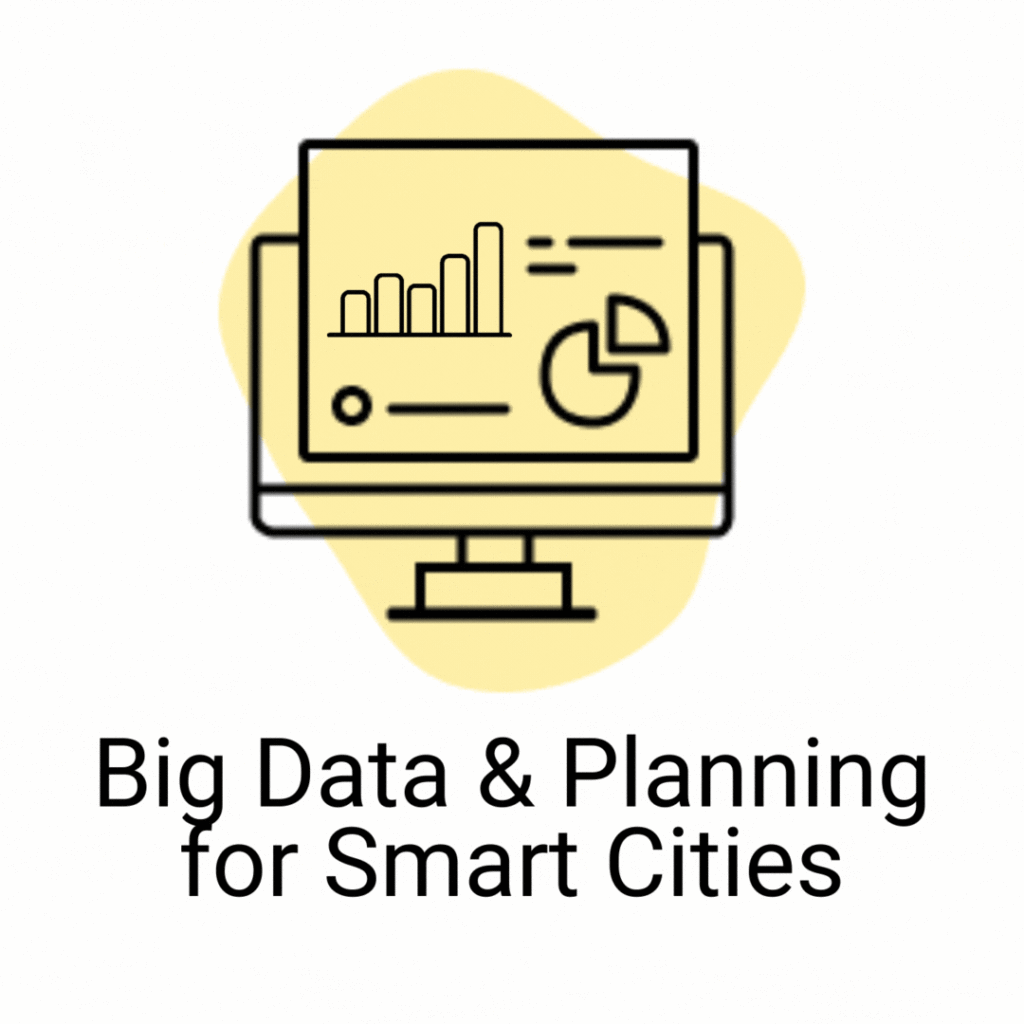
Automated Lane Change and Robust Safety
PI: Zhong-Ping Jiang
Co-PI: Kaan Ozbay
This collaborative research project aims to take a significant step forward to develop innovative solutions for autonomous lane change maneuvers, and builds on our prior work on lane keeping and lane changing for automated vehicles.
This project aims to achieve three major objectives: (1) integrating reinforcement learning and (control) barrier functions to address safety-oriented constraints; (2) developing robustness analysis and robust redesign for connected and autonomous vehicles in the presence of uncertainties and time delay; and (3) validating the proposed lane changing control algorithms with real-world trajectory data and SUMO testing under different environments in the presence of different vehicle mixes and driver uncertainties.
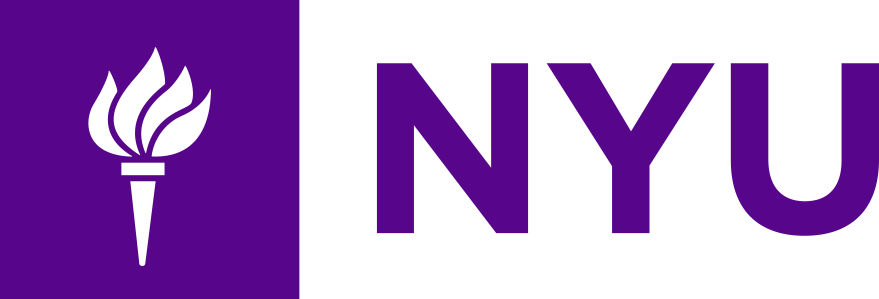

Digital Twin Technologies Towards Understanding the Interactions between Transportation and other Civil Infrastructure Systems
PI: Ruimin Ke
Co-PI: Jeffrey Weidner, Adeeba Raheem, Kelvin Cheu
In phase 1 of this project, the team built a digital shadow of University of Texas – El Paso campus civil infrastructure and visualized impacts of the construction project schedule on the surrounding transportation infrastructure.
In phase 2, this project is focused on three aspects of expansion: (a) extending the digital twin (DT) model from a building to multiple representative locations on campus; (b) developing a Smart Living Lab (SLL), which enables live data streams from three selected sites on campus via sensor instrumentation, data communication, and database design; and (c) demonstrating a working prototype of digital twin, integrated with the digital model (DM) for real-time scenarios analysis, infrastructure interactions, and decision-making.

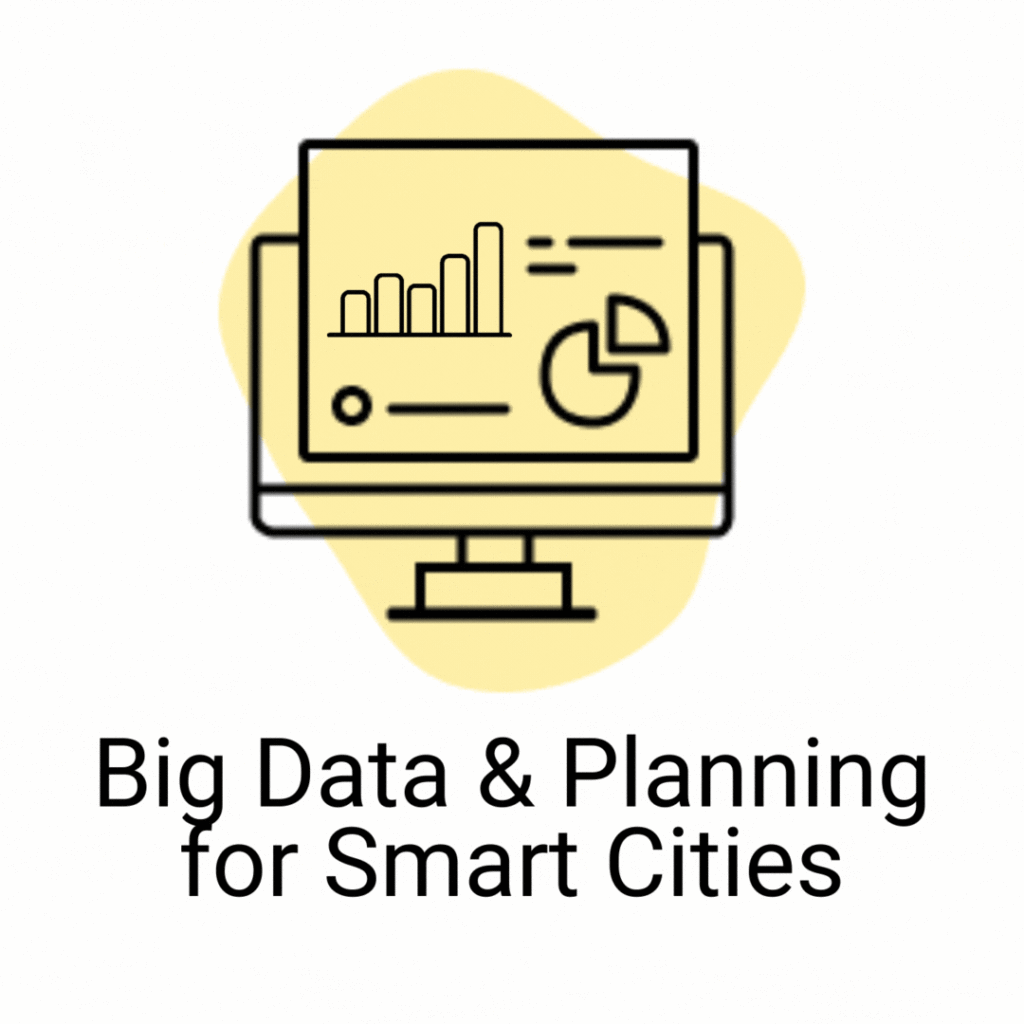
Developing a Framework to Optimize FloodNet Sensor Deployments Around NYC for Equitable and Impact-based Hyper-local Street-level Flood Monitoring and Data Collection
PI: Luis Ceferino
Co-PIs: Andrea Silverman, Elizabeth Henaff, Charlie Mydlarz, Tega Brain
This continuation of the FloodNet project will build a framework to optimize and prioritize locations for sensor deployment and measurement of hyper-localflooding in New York City (NYC). The research team will build a risk-informed digital twin of NYC, integrating multiple data streams and risk metrics.
Risk metrics will be chosen based on impacts – e.g., fatalities or subway and street disruptions – rather than relying on direct hazard metrics, such as flood depth and extent, alone. The digital twin will also utilize explicit equity risk metrics, such as neighborhoods at disproportionally higher risk.
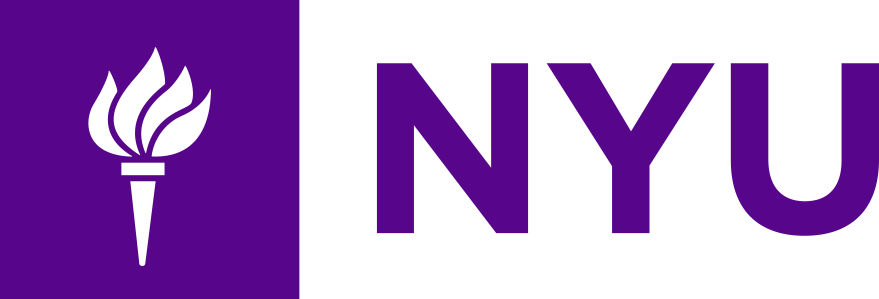

Work Zone Safety: Virtual reality -based traffic co-simulation platform for workforce training and pedestrian behavior analysis
PI: Semiha Ergan
Co-PI: Kaan Ozbay
Since 2006, fatalities in US roadway construction work zones have reached their highest level, with a significant fraction involving struck-by vehicles and workers.
This project aims to address this pressing challenge by (a) developing a virtual reality (VR) traffic co-simulation platform for enabling immersive training of construction workers under realistic exposures to dangers, and (b) calibrating modality of alarms (e.g.,frequency, sound, vibration and their variations) to promote safe behaviors when workers are exposed to different wearable alarm systems.
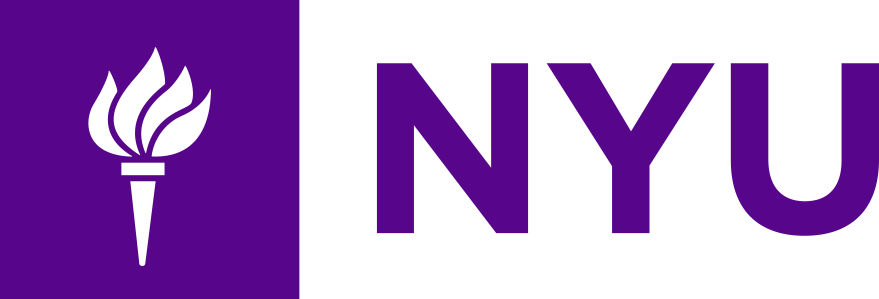

Evaluation of Integrated Overweight Enforcement System using High Accuracy WIM System and Non-Proprietary ALPR System
PI: Hani Nassif
Co-PI: Kaan Ozbay
The team has been collaborating with NYCDOT to establish a testbed at the north portion of the triple cantilever of the BQE to understand the site-specific overweight truck characteristics (local or interstate trucks, truck type, tonnage, etc.) and automated license plate recognition (ALPR) system to identify these overweight trucks.
However, this testbed is limited to one lane only and is not able to capture the entire triple cantilever of the BQE. Therefore, the team will work with NYCDOT to establish another testbed at the south portion of the BQE, to be equipped with WIM and ALPR systems for all six (6) lanes. Moreover , the team will work with NYCDOT to develop a plan to expand the network of WIM stations across NYC for future implementation.
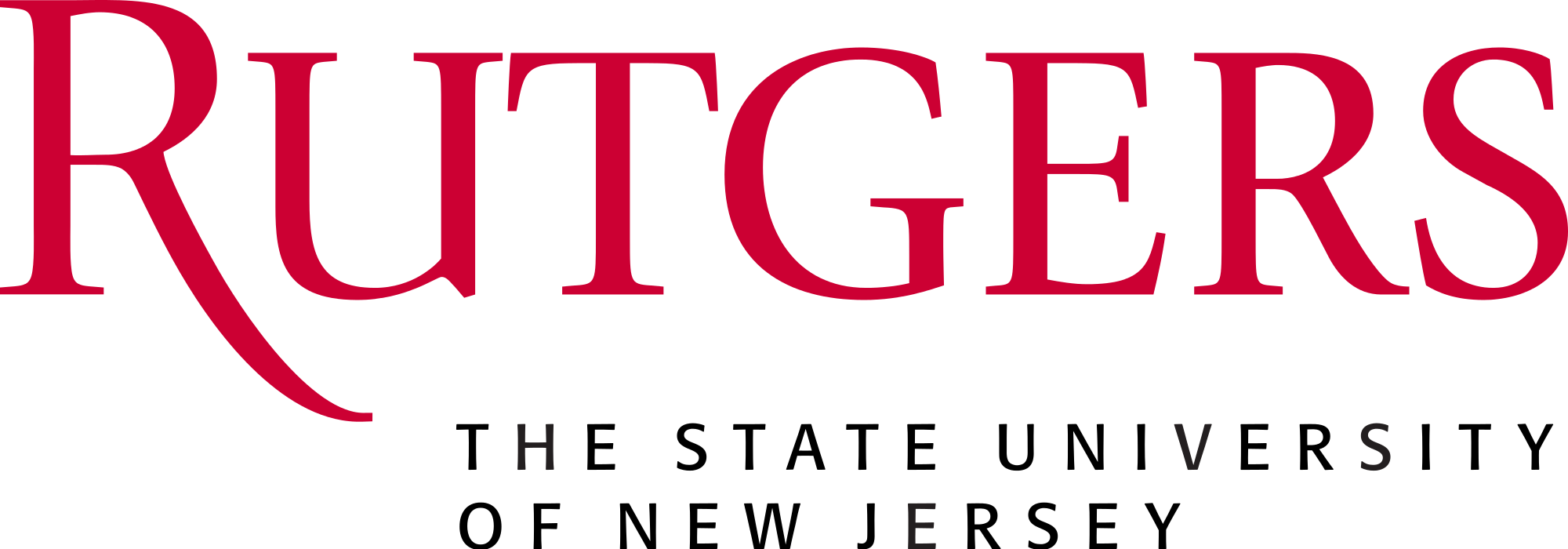
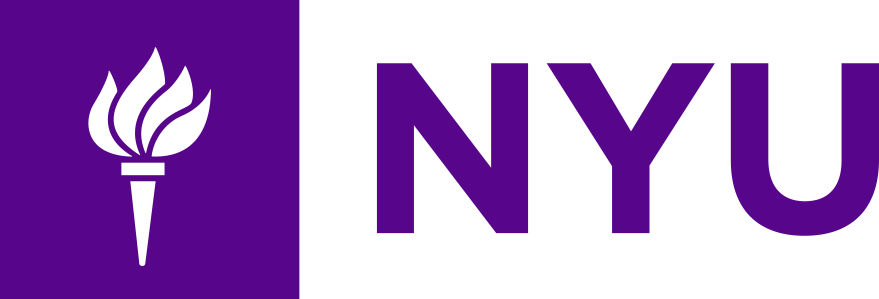

Exploring Cost-effective Computer Vision Solutions for Smart Transportation Systems
PI: Jingqin Gao
Co-PI: Kaan Ozbay
This project is focused on developing a deep learning-based data acquisition and analytics tool using vision-based sensors (i.e., cameras) to understand cities with machine eyes. The team will assess the maturity of various smart city applications using computer vision and object detection (e.g., pedestrian detection, work zone identification, curb lane usage, connected and automated vehicles) as well as the needs of the local agencies.
The goal is to demonstrate the cost-effectiveness of the computer vision technology to generate new stream of mobility data and provide support for planning and operational strategies, especially with an emphasis on utilizing both existing transportation infrastructure and emerging probe and CAVs.
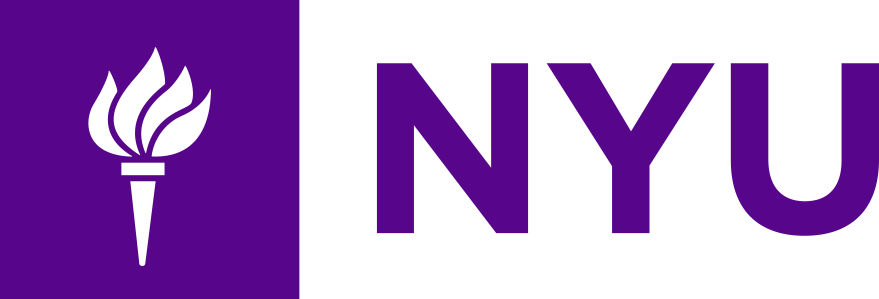
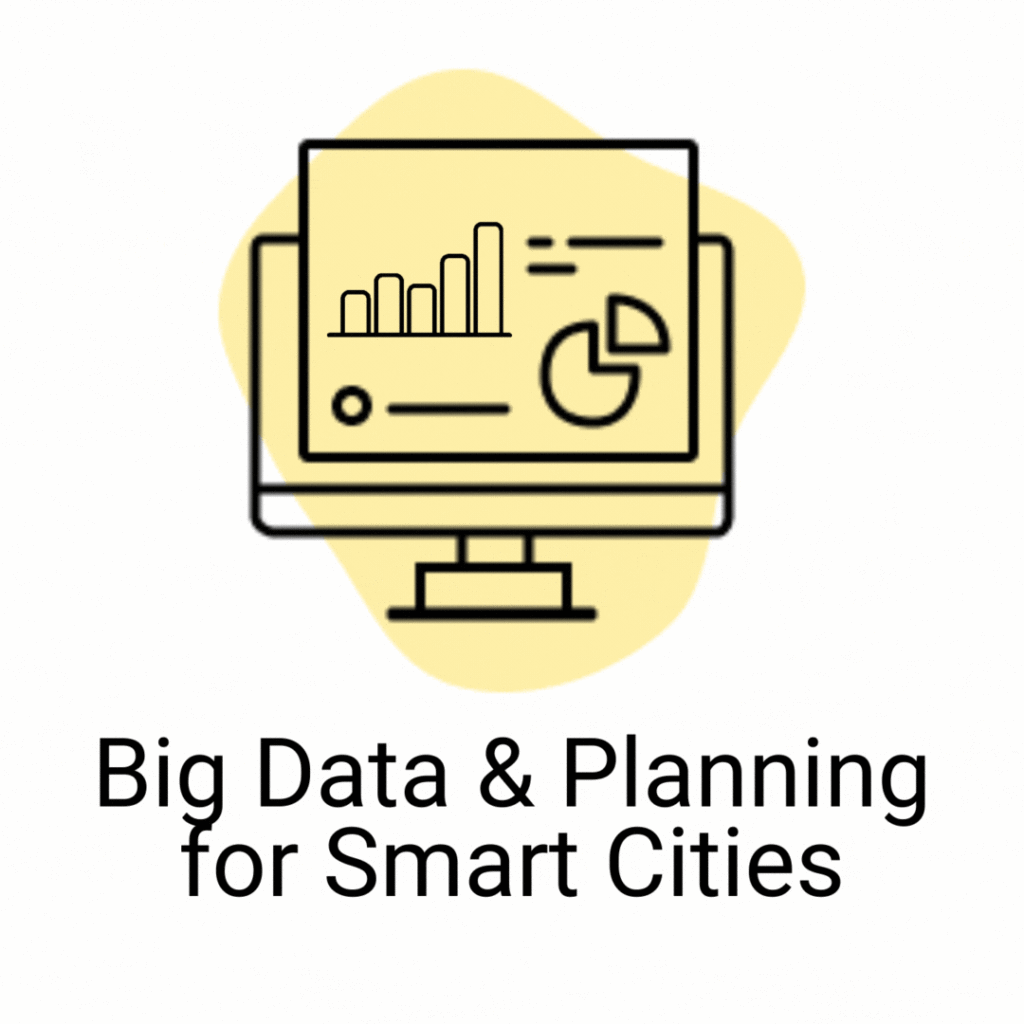